Experimental Design: Types, Examples & Methods
Saul McLeod, PhD
Editor-in-Chief for Simply Psychology
BSc (Hons) Psychology, MRes, PhD, University of Manchester
Saul McLeod, PhD., is a qualified psychology teacher with over 18 years of experience in further and higher education. He has been published in peer-reviewed journals, including the Journal of Clinical Psychology.
Learn about our Editorial Process
Olivia Guy-Evans, MSc
Associate Editor for Simply Psychology
BSc (Hons) Psychology, MSc Psychology of Education
Olivia Guy-Evans is a writer and associate editor for Simply Psychology. She has previously worked in healthcare and educational sectors.
On This Page:
Experimental design refers to how participants are allocated to different groups in an experiment. Types of design include repeated measures, independent groups, and matched pairs designs.
Probably the most common way to design an experiment in psychology is to divide the participants into two groups, the experimental group and the control group, and then introduce a change to the experimental group, not the control group.
The researcher must decide how he/she will allocate their sample to the different experimental groups. For example, if there are 10 participants, will all 10 participants participate in both groups (e.g., repeated measures), or will the participants be split in half and take part in only one group each?
Three types of experimental designs are commonly used:

1. Independent Measures
Independent measures design, also known as between-groups , is an experimental design where different participants are used in each condition of the independent variable. This means that each condition of the experiment includes a different group of participants.
This should be done by random allocation, ensuring that each participant has an equal chance of being assigned to one group.
Independent measures involve using two separate groups of participants, one in each condition. For example:
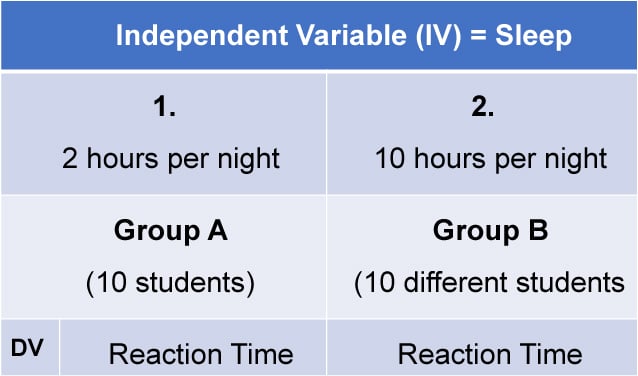
- Con : More people are needed than with the repeated measures design (i.e., more time-consuming).
- Pro : Avoids order effects (such as practice or fatigue) as people participate in one condition only. If a person is involved in several conditions, they may become bored, tired, and fed up by the time they come to the second condition or become wise to the requirements of the experiment!
- Con : Differences between participants in the groups may affect results, for example, variations in age, gender, or social background. These differences are known as participant variables (i.e., a type of extraneous variable ).
- Control : After the participants have been recruited, they should be randomly assigned to their groups. This should ensure the groups are similar, on average (reducing participant variables).
2. Repeated Measures Design
Repeated Measures design is an experimental design where the same participants participate in each independent variable condition. This means that each experiment condition includes the same group of participants.
Repeated Measures design is also known as within-groups or within-subjects design .
- Pro : As the same participants are used in each condition, participant variables (i.e., individual differences) are reduced.
- Con : There may be order effects. Order effects refer to the order of the conditions affecting the participants’ behavior. Performance in the second condition may be better because the participants know what to do (i.e., practice effect). Or their performance might be worse in the second condition because they are tired (i.e., fatigue effect). This limitation can be controlled using counterbalancing.
- Pro : Fewer people are needed as they participate in all conditions (i.e., saves time).
- Control : To combat order effects, the researcher counter-balances the order of the conditions for the participants. Alternating the order in which participants perform in different conditions of an experiment.
Counterbalancing
Suppose we used a repeated measures design in which all of the participants first learned words in “loud noise” and then learned them in “no noise.”
We expect the participants to learn better in “no noise” because of order effects, such as practice. However, a researcher can control for order effects using counterbalancing.
The sample would be split into two groups: experimental (A) and control (B). For example, group 1 does ‘A’ then ‘B,’ and group 2 does ‘B’ then ‘A.’ This is to eliminate order effects.
Although order effects occur for each participant, they balance each other out in the results because they occur equally in both groups.
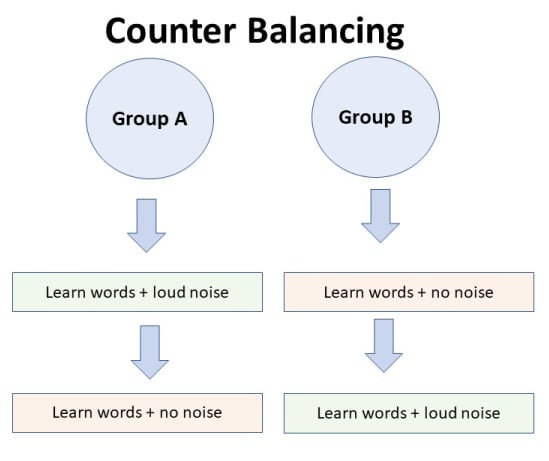
3. Matched Pairs Design
A matched pairs design is an experimental design where pairs of participants are matched in terms of key variables, such as age or socioeconomic status. One member of each pair is then placed into the experimental group and the other member into the control group .
One member of each matched pair must be randomly assigned to the experimental group and the other to the control group.
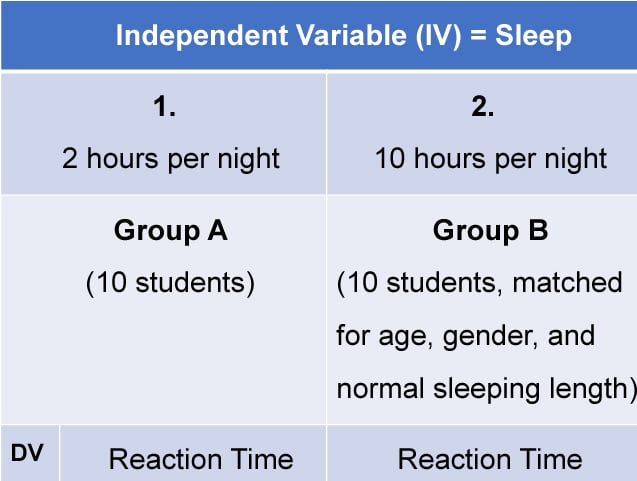
- Con : If one participant drops out, you lose 2 PPs’ data.
- Pro : Reduces participant variables because the researcher has tried to pair up the participants so that each condition has people with similar abilities and characteristics.
- Con : Very time-consuming trying to find closely matched pairs.
- Pro : It avoids order effects, so counterbalancing is not necessary.
- Con : Impossible to match people exactly unless they are identical twins!
- Control : Members of each pair should be randomly assigned to conditions. However, this does not solve all these problems.
Experimental design refers to how participants are allocated to an experiment’s different conditions (or IV levels). There are three types:
1. Independent measures / between-groups : Different participants are used in each condition of the independent variable.
2. Repeated measures /within groups : The same participants take part in each condition of the independent variable.
3. Matched pairs : Each condition uses different participants, but they are matched in terms of important characteristics, e.g., gender, age, intelligence, etc.
Learning Check
Read about each of the experiments below. For each experiment, identify (1) which experimental design was used; and (2) why the researcher might have used that design.
1 . To compare the effectiveness of two different types of therapy for depression, depressed patients were assigned to receive either cognitive therapy or behavior therapy for a 12-week period.
The researchers attempted to ensure that the patients in the two groups had similar severity of depressed symptoms by administering a standardized test of depression to each participant, then pairing them according to the severity of their symptoms.
2 . To assess the difference in reading comprehension between 7 and 9-year-olds, a researcher recruited each group from a local primary school. They were given the same passage of text to read and then asked a series of questions to assess their understanding.
3 . To assess the effectiveness of two different ways of teaching reading, a group of 5-year-olds was recruited from a primary school. Their level of reading ability was assessed, and then they were taught using scheme one for 20 weeks.
At the end of this period, their reading was reassessed, and a reading improvement score was calculated. They were then taught using scheme two for a further 20 weeks, and another reading improvement score for this period was calculated. The reading improvement scores for each child were then compared.
4 . To assess the effect of the organization on recall, a researcher randomly assigned student volunteers to two conditions.
Condition one attempted to recall a list of words that were organized into meaningful categories; condition two attempted to recall the same words, randomly grouped on the page.
Experiment Terminology
Ecological validity.
The degree to which an investigation represents real-life experiences.
Experimenter effects
These are the ways that the experimenter can accidentally influence the participant through their appearance or behavior.
Demand characteristics
The clues in an experiment lead the participants to think they know what the researcher is looking for (e.g., the experimenter’s body language).
Independent variable (IV)
The variable the experimenter manipulates (i.e., changes) is assumed to have a direct effect on the dependent variable.
Dependent variable (DV)
Variable the experimenter measures. This is the outcome (i.e., the result) of a study.
Extraneous variables (EV)
All variables which are not independent variables but could affect the results (DV) of the experiment. Extraneous variables should be controlled where possible.
Confounding variables
Variable(s) that have affected the results (DV), apart from the IV. A confounding variable could be an extraneous variable that has not been controlled.
Random Allocation
Randomly allocating participants to independent variable conditions means that all participants should have an equal chance of taking part in each condition.
The principle of random allocation is to avoid bias in how the experiment is carried out and limit the effects of participant variables.
Order effects
Changes in participants’ performance due to their repeating the same or similar test more than once. Examples of order effects include:
(i) practice effect: an improvement in performance on a task due to repetition, for example, because of familiarity with the task;
(ii) fatigue effect: a decrease in performance of a task due to repetition, for example, because of boredom or tiredness.

- Number System and Arithmetic
- Probability
- Mensuration
- Trigonometry
- Mathematics
Experimental Design
Experimental design is reviewed as an important part of the research methodology with an implication for the confirmation and reliability of the scientific studies. This is the scientific, logical and planned way of arranging tests and how they may be conducted so that hypotheses can be tested with the possibility of arriving at some conclusions. It refers to a procedure followed in order to control variables and conditions that may influence the outcome of a given study to reduce bias as well as improve the effectiveness of data collection and subsequently the quality of the results.
What is Experimental Design?
Experimental design simply refers to the strategy that is employed in conducting experiments to test hypotheses and arrive at valid conclusions. The process comprises firstly, the formulation of research questions, variable selection, specifications of the conditions for the experiment, and a protocol for data collection and analysis. The importance of experimental design can be seen through its potential to prevent bias, reduce variability, and increase the precision of results in an attempt to achieve high internal validity of studies. By using experimental design, the researchers can generate valid results which can be generalized in other settings which helps the advancement of knowledge in various fields.
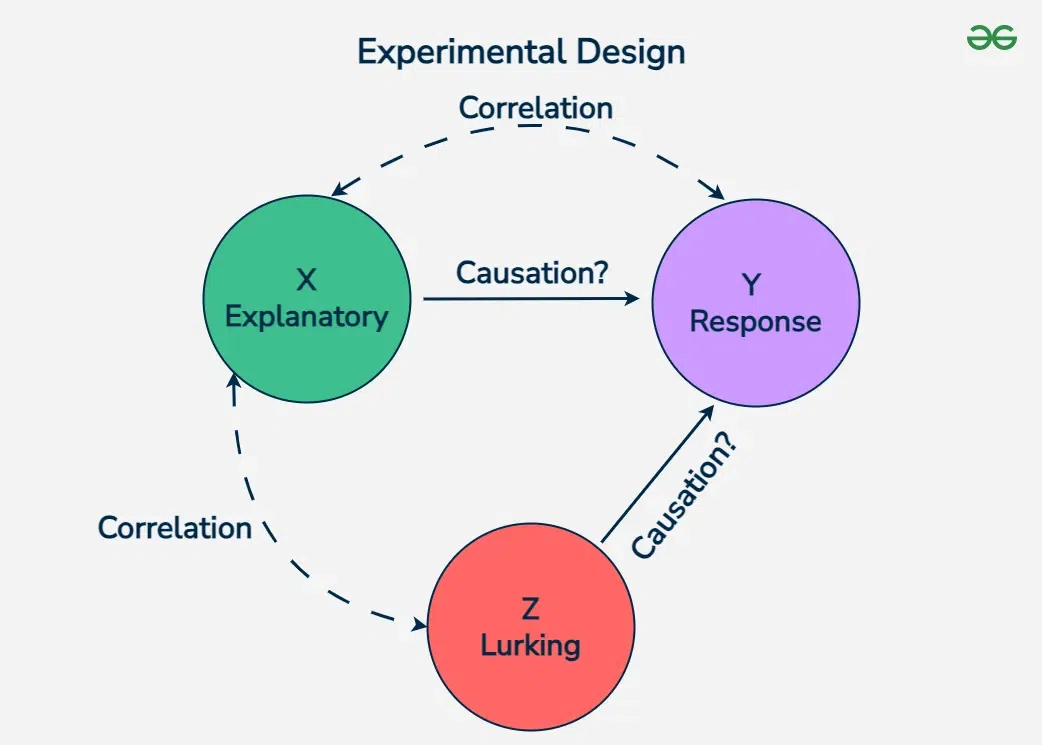
Definition of Experimental Design
Experimental design is a systematic method of implementing experiments in which one can manipulate variables in a structured way in order to analyze hypotheses and draw outcomes based on empirical evidence.
Types of Experimental Design
Experimental design encompasses various approaches to conducting research studies, each tailored to address specific research questions and objectives. The primary types of experimental design include:
Pre-experimental Research Design
- True Experimental Research Design
- Quasi-Experimental Research Design
Statistical Experimental Design
A preliminary approach where groups are observed after implementing cause and effect factors to determine the need for further investigation. It is often employed when limited information is available or when researchers seek to gain initial insights into a topic. Pre-experimental designs lack random assignment and control groups, making it difficult to establish causal relationships.
Classifications:
- One-Shot Case Study
- One-Group Pretest-Posttest Design
- Static-Group Comparison
True-experimental Research Design
The true-experimental research design involves the random assignment of participants to experimental and control groups to establish cause-and-effect relationships between variables. It is used to determine the impact of an intervention or treatment on the outcome of interest. True-experimental designs satisfy the following factors:
Factors to Satisfy:
- Random Assignment
- Control Group
- Experimental Group
- Pretest-Posttest Measures
Quasi-Experimental Design
A quasi-experimental design is an alternative to the true-experimental design when the random assignment of participants to the groups is not possible or desirable. It allows for comparisons between groups without random assignment, providing valuable insights into causal relationships in real-world settings. Quasi-experimental designs are used typically in conditions wherein the random assignment of the participants cannot be done or it may not be ethical, for example, an educational or community-based intervention.
Statistical experimental design, also known as design of experiments (DOE), is a branch of statistics that focuses on planning, conducting, analyzing, and interpreting controlled tests to evaluate the factors that may influence a particular outcome or process. The primary goal is to determine cause-and-effect relationships and to identify the optimal conditions for achieving desired results. The detailed is discussed below:
Design of Experiments: Goals & Settings
The goals and settings for design of experiments are as follows:
- Identifying Research Objectives: Clearly defining the goals and hypotheses of the experiment is crucial for designing an effective study.
- Selecting Appropriate Variables: Determining the independent, dependent, and control variables based on the research question.
- Considering Experimental Conditions: Identifying the settings and constraints under which the experiment will be conducted.
- Ensuring Validity and Reliability: Designing the experiment to minimize threats to internal and external validity.
Developing an Experimental Design
Developing an experimental design involves a systematic process of planning and structuring the study to achieve the research objectives. Here are the key steps:
- Define the research question and hypotheses
- Identify the independent and dependent variables
- Determine the experimental conditions and treatments
- Select the appropriate experimental design (e.g., completely randomized, randomized block, factorial)
- Determine the sample size and sampling method
- Establish protocols for data collection and analysis
- Conduct a pilot study to test the feasibility and refine the design
- Implement the experiment and collect data
- Analyze the data using appropriate statistical methods
- Interpret the results and draw conclusions
Preplanning, Defining, and Operationalizing for Design of Experiments
Preplanning, defining, and operationalizing are crucial steps in the design of experiments. Preplanning involves identifying the research objectives, selecting variables, and determining the experimental conditions. Defining refers to clearly stating the research question, hypotheses, and operational definitions of the variables. Operationalizing involves translating the conceptual definitions into measurable terms and establishing protocols for data collection.
For example, in a study investigating the effect of different fertilizers on plant growth, the researcher would preplan by selecting the independent variable (fertilizer type), dependent variable (plant height), and control variables (soil type, sunlight exposure). The research question would be defined as "Does the type of fertilizer affect the height of plants?" The operational definitions would include specific methods for measuring plant height and applying the fertilizers.
Randomized Block Design
Randomized block design is an experimental approach where subjects or units are grouped into blocks based on a known source of variability, such as location, time, or individual characteristics. The treatments are then randomly assigned to the units within each block. This design helps control for confounding factors, reduce experimental error, and increase the precision of estimates. By blocking, researchers can account for systematic differences between groups and focus on the effects of the treatments being studied
Consider a study investigating the effectiveness of two teaching methods (A and B) on student performance. The steps involved in a randomized block design would include:
- Identifying blocks based on student ability levels.
- Randomly assigning students within each block to either method A or B.
- Conducting the teaching interventions.
- Analyzing the results within each block to account for variability.
Completely Randomized Design
A completely randomized design is a straightforward experimental approach where treatments are randomly assigned to experimental units without any specific blocking. This design is suitable when there are no known sources of variability that need to be controlled for. In a completely randomized design, all units have an equal chance of receiving any treatment, and the treatments are distributed independently. This design is simple to implement and analyze but may be less efficient than a randomized block design when there are known sources of variability
Between-Subjects vs Within-Subjects Experimental Designs
Here is a detailed comparison among Between-Subject and Within-Subject is tabulated below:
Design of Experiments Examples
The examples of design experiments are as follows:
Between-Subjects Design Example:
In a study comparing the effectiveness of two teaching methods on student performance, one group of students (Group A) is taught using Method 1, while another group (Group B) is taught using Method 2. The performance of both groups is then compared to determine the impact of the teaching methods on student outcomes.
Within-Subjects Design Example:
In a study assessing the effects of different exercise routines on fitness levels, each participant undergoes all exercise routines over a period of time. Participants' fitness levels are measured before and after each routine to evaluate the impact of the exercises on their fitness levels.
Application of Experimental Design
The applications of Experimental design are as follows:
- Product Testing: Experimental design is used to evaluate the effectiveness of new products or interventions.
- Medical Research: It helps in testing the efficacy of treatments and interventions in controlled settings.
- Agricultural Studies: Experimental design is crucial in testing new farming techniques or crop varieties.
- Psychological Experiments: It is employed to study human behavior and cognitive processes.
- Quality Control: Experimental design aids in optimizing processes and improving product quality.
In scientific research, experimental design is a crucial procedure that helps to outline an effective strategy for carrying out a meaningful experiment and making correct conclusions. This means that through proper control and coordination in conducting experiments, increased reliability and validity can be attained, and expansion of knowledge can take place generally across various fields. Using proper experimental design principles is crucial in ensuring that the experimental outcomes are impactful and valid.
Also, Check
- What is Hypothesis
- Null Hypothesis
- Real-life Applications of Hypothesis Testing
FAQs on Experimental Design
What is experimental design in math.
Experimental design refers to the aspect of planning experiments to gather data, decide the way in which to control the variable and draw sensible conclusions from the outcomes.
What are the advantages of the experimental method in math?
The advantages of the experimental method include control of variables, establishment of cause-and-effector relationship and use of statistical tools for proper data analysis.
What is the main purpose of experimental design?
The goal of experimental design is to describe the nature of variables and examine how changes in one or more variables impact the outcome of the experiment.
What are the limitations of experimental design?
Limitations include potential biases, the complexity of controlling all variables, ethical considerations, and the fact that some experiments can be costly or impractical.
What are the statistical tools used in experimental design?
Statistical tools utilized include ANOVA, regression analysis, t-tests, chi-square tests and factorial designs to conduct scientific research.
Similar Reads
- jQuery getJSON() Method In this article, we will learn about the getJSON() method in jQuery, along with understanding their implementation through the example. jQuery is an open-source JavaScript library that simplifies the interactions between an HTML/CSS document, It is widely famous for its philosophy of “Write less, do 2 min read
- How to open JSON file ? In this article, we will open the JSON file using JavaScript. JSON stands for JavaScript Object Notation. It is basically a format for structuring data. The JSON format is a text-based format to represent the data in form of a JavaScript object. Approach: Create a JSON file, add data in that JSON f 2 min read
- How to open json file ? A JSON (JavaScript Object Notation) file is a standard format used to store and exchange data. Initially, JSON was primarily used for data transfer between web applications and servers, but its usage has expanded significantly. Today, JSON files are used for various purposes, including data backups 3 min read
- JSON Schema JSON Schema is a content specification language used for validating the structure of a JSON data.It helps you specify the objects and what values are valid inside the object's properties. JSON schema is useful in offering clear, human-readable, and machine-readable documentation. Structure of a JSON 2 min read
- What is JSON text ? The Javascript Object Notation(JSON) is used to transfer or exchange information on the internet. JSON is just the plain text written as a javascript object. There exist several key-value pairs which represent some useful information. The important thing to keep in mind is, although the JavaScript c 3 min read
- ES6 Merge Objects In this article, we are going to learn how to merge two objects in JavaScript. We can merge two JavaScript Objects in ES6 by using the two popular methods. The methods are listed below: Object.assign() methodObject spread syntax method Using Object.assign() method: Using this method, we can merge tw 3 min read
- How To Escape Strings in JSON? JSON (JavaScript Object Notation) is a popular data format that is widely used in APIs, configuration files, and data storage. While JSON is straightforward, handling special characters within strings requires some extra care. Certain characters must be escaped to be valid within a JSON string. Tabl 3 min read
- How to Handle Newlines in JSON? Handling newlines in JSON is essential for maintaining data integrity when dealing with multiline text. JSON, being a text-based format, requires special consideration for newlines to ensure that text appears as intended across different platforms. Proper handling of newlines ensures consistent and 2 min read
- Node.js querystring.encode() Function The querystring.encode() method is used to produce a URL query string from the given object that contains the key-value pairs. The method iterates through the object’s own properties to generate the query string. It can serialize a single or an array of strings, numbers, and boolean. Any other types 2 min read
- Rename Object Key in JavaScript In JavaScript, objects are used to store the collection of various data. It is a collection of properties. A property is a "key: value" pair where Keys are known as 'property name' and are used to identify values. Since JavaScript doesn't provide an inbuilt function to rename an object key. So we wi 4 min read
- String in JavaScript String is used to representing the sequence of characters. They are made up of a list of characters, which is essentially just an "array of characters". It can hold the data and manipulate the data which can be represented in the text form. We can perform different operations on strings like checkin 3 min read
- Add Nested JSON Object in Postman Postman is a helpful tool for testing APIs and working with JSON. It lets you add nested objects to existing JSON. JSON is a simple data format for both humans and machines. Postman also lets developers send requests and view responses, which is really useful for working with JSON data. These are th 2 min read
- Read, Write and Parse JSON using Python JSON is a lightweight data format for data interchange that can be easily read and written by humans, and easily parsed and generated by machines. It is a complete language-independent text format. To work with JSON data, Python has a built-in package called JSON. Example of JSON String s = '{"id":0 4 min read
- Deserializing a JSON into a JavaScript object Deserializing a JSON into a JavaScript object refers to the process of converting a JSON (JavaScript Object Notation) formatted string into a native JavaScript object. This allows developers to work with the data in JavaScript by accessing its properties and methods directly. JavaScript Object Notat 2 min read
- JavaScript JSON parse() Method The JSON.parse() method is used to convert a JSON string into a JavaScript object. It’s become important when dealing with data in JSON format, interacting with APIs, or storing data in the browser. It converts a JSON string into a JavaScript object.Throws a SyntaxError if the input string is not va 4 min read
- How to Receive JSON Data at Server Side ? JavaScript Object Notation (JSON) is a data transferring format used to send data to or from the server. It is commonly utilized in API integration due to its benefits and simplicity. In this example, we will utilize XML HttpRequest to deliver data to the server. Frontend: We will use a simple form 2 min read
- How to Convert String to Variable Name in JavaScript? In JavaScript, if we consider the variable names as string values, it is difficult to transform a variable’s name into a string. However, this problem has been handled in many ways for example, static properties of objects, eval(), and modern ways such as Map can be used to achieve the essential eff 3 min read
- How to Pass Object as Parameter in JavaScript ? We'll see how to Pass an object as a Parameter in JavaScript. We can pass objects as parameters to functions just like we do with any other data type. passing objects as parameters to functions allows us to manipulate their properties within the function. These are the following approaches: Table of 3 min read
- JavaScript JSON Parser JSON (JavaScript Object Notation) is a popular lightweight data exchange format for sending data between a server and a client, or across various systems. JSON data is parsed and interpreted using a software component or library called a JSON parser. Through the JSON parsing process, a JSON string i 3 min read
- School Learning
- Math-Statistics
Improve your Coding Skills with Practice
What kind of Experience do you want to share?
- Privacy Policy

Home » Experimental Design – Types, Methods, Guide
Experimental Design – Types, Methods, Guide
Table of Contents
Experimental design is a structured approach used to conduct scientific experiments. It enables researchers to explore cause-and-effect relationships by controlling variables and testing hypotheses. This guide explores the types of experimental designs, common methods, and best practices for planning and conducting experiments.

Experimental Design
Experimental design refers to the process of planning a study to test a hypothesis, where variables are manipulated to observe their effects on outcomes. By carefully controlling conditions, researchers can determine whether specific factors cause changes in a dependent variable.
Key Characteristics of Experimental Design :
- Manipulation of Variables : The researcher intentionally changes one or more independent variables.
- Control of Extraneous Factors : Other variables are kept constant to avoid interference.
- Randomization : Subjects are often randomly assigned to groups to reduce bias.
- Replication : Repeating the experiment or having multiple subjects helps verify results.
Purpose of Experimental Design
The primary purpose of experimental design is to establish causal relationships by controlling for extraneous factors and reducing bias. Experimental designs help:
- Test Hypotheses : Determine if there is a significant effect of independent variables on dependent variables.
- Control Confounding Variables : Minimize the impact of variables that could distort results.
- Generate Reproducible Results : Provide a structured approach that allows other researchers to replicate findings.
Types of Experimental Designs
Experimental designs can vary based on the number of variables, the assignment of participants, and the purpose of the experiment. Here are some common types:
1. Pre-Experimental Designs
These designs are exploratory and lack random assignment, often used when strict control is not feasible. They provide initial insights but are less rigorous in establishing causality.
- Example : A training program is provided, and participants’ knowledge is tested afterward, without a pretest.
- Example : A group is tested on reading skills, receives instruction, and is tested again to measure improvement.
2. True Experimental Designs
True experiments involve random assignment of participants to control or experimental groups, providing high levels of control over variables.
- Example : A new drug’s efficacy is tested with patients randomly assigned to receive the drug or a placebo.
- Example : Two groups are observed after one group receives a treatment, and the other receives no intervention.
3. Quasi-Experimental Designs
Quasi-experiments lack random assignment but still aim to determine causality by comparing groups or time periods. They are often used when randomization isn’t possible, such as in natural or field experiments.
- Example : Schools receive different curriculums, and students’ test scores are compared before and after implementation.
- Example : Traffic accident rates are recorded for a city before and after a new speed limit is enforced.

4. Factorial Designs
Factorial designs test the effects of multiple independent variables simultaneously. This design is useful for studying the interactions between variables.
- Example : Studying how caffeine (variable 1) and sleep deprivation (variable 2) affect memory performance.
- Example : An experiment studying the impact of age, gender, and education level on technology usage.
5. Repeated Measures Design
In repeated measures designs, the same participants are exposed to different conditions or treatments. This design is valuable for studying changes within subjects over time.
- Example : Measuring reaction time in participants before, during, and after caffeine consumption.
- Example : Testing two medications, with each participant receiving both but in a different sequence.
Methods for Implementing Experimental Designs
- Purpose : Ensures each participant has an equal chance of being assigned to any group, reducing selection bias.
- Method : Use random number generators or assignment software to allocate participants randomly.
- Purpose : Prevents participants or researchers from knowing which group (experimental or control) participants belong to, reducing bias.
- Method : Implement single-blind (participants unaware) or double-blind (both participants and researchers unaware) procedures.
- Purpose : Provides a baseline for comparison, showing what would happen without the intervention.
- Method : Include a group that does not receive the treatment but otherwise undergoes the same conditions.
- Purpose : Controls for order effects in repeated measures designs by varying the order of treatments.
- Method : Assign different sequences to participants, ensuring that each condition appears equally across orders.
- Purpose : Ensures reliability by repeating the experiment or including multiple participants within groups.
- Method : Increase sample size or repeat studies with different samples or in different settings.
Steps to Conduct an Experimental Design
- Clearly state what you intend to discover or prove through the experiment. A strong hypothesis guides the experiment’s design and variable selection.
- Independent Variable (IV) : The factor manipulated by the researcher (e.g., amount of sleep).
- Dependent Variable (DV) : The outcome measured (e.g., reaction time).
- Control Variables : Factors kept constant to prevent interference with results (e.g., time of day for testing).
- Choose a design type that aligns with your research question, hypothesis, and available resources. For example, an RCT for a medical study or a factorial design for complex interactions.
- Randomly assign participants to experimental or control groups. Ensure control groups are similar to experimental groups in all respects except for the treatment received.
- Randomize the assignment and, if possible, apply blinding to minimize potential bias.
- Follow a consistent procedure for each group, collecting data systematically. Record observations and manage any unexpected events or variables that may arise.
- Use appropriate statistical methods to test for significant differences between groups, such as t-tests, ANOVA, or regression analysis.
- Determine whether the results support your hypothesis and analyze any trends, patterns, or unexpected findings. Discuss possible limitations and implications of your results.
Examples of Experimental Design in Research
- Medicine : Testing a new drug’s effectiveness through a randomized controlled trial, where one group receives the drug and another receives a placebo.
- Psychology : Studying the effect of sleep deprivation on memory using a within-subject design, where participants are tested with different sleep conditions.
- Education : Comparing teaching methods in a quasi-experimental design by measuring students’ performance before and after implementing a new curriculum.
- Marketing : Using a factorial design to examine the effects of advertisement type and frequency on consumer purchase behavior.
- Environmental Science : Testing the impact of a pollution reduction policy through a time series design, recording pollution levels before and after implementation.
Experimental design is fundamental to conducting rigorous and reliable research, offering a systematic approach to exploring causal relationships. With various types of designs and methods, researchers can choose the most appropriate setup to answer their research questions effectively. By applying best practices, controlling variables, and selecting suitable statistical methods, experimental design supports meaningful insights across scientific, medical, and social research fields.
- Campbell, D. T., & Stanley, J. C. (1963). Experimental and Quasi-Experimental Designs for Research . Houghton Mifflin Company.
- Shadish, W. R., Cook, T. D., & Campbell, D. T. (2002). Experimental and Quasi-Experimental Designs for Generalized Causal Inference . Houghton Mifflin.
- Fisher, R. A. (1935). The Design of Experiments . Oliver and Boyd.
- Field, A. (2013). Discovering Statistics Using IBM SPSS Statistics . Sage Publications.
- Cohen, J. (1988). Statistical Power Analysis for the Behavioral Sciences . Routledge.
About the author
Muhammad Hassan
Researcher, Academic Writer, Web developer
You may also like

Survey Research – Types, Methods, Examples

Research Methods – Types, Examples and Guide

Transformative Design – Methods, Types, Guide

Triangulation in Research – Types, Methods and...

Exploratory Research – Types, Methods and...

Qualitative Research Methods
Experimental Design: The Building Blocks of Reliable Research
Unleash the potential of well-crafted experimental design to steer the course of robust and enlightening scientific breakthroughs.
When it comes to scientific research, figuring out why things happen is essential. This is where experimental design becomes indispensable, helping researchers uncover the secrets behind cause-and-effect relationships. By carefully planning experiments, collecting precise data, and analyzing them thoughtfully, experimental design gives researchers the tools to identify and understand how different things are connected. In this article, we’ll explore the field of experimental design, understand its importance, purpose, and the different methods used in various areas of study.
What Is Experimental Design?
Experimental design is a systematic approach to planning, conducting, and analyzing experiments to identify and understand causal relationships between variables. It involves carefully designing the experiment to control for potential confounding factors and to ensure that data collection efforts effectively address research questions and hypotheses. Experimental design encompasses the selection of factors and treatments, the assignment of participants or subjects to different conditions, and the collection and analysis of data to draw meaningful conclusions. By employing various experimental designs, such as completely randomized, randomized block, and observational Studies, researchers can enhance the validity and reliability of their findings.
Types Of Experimental Design
Experimental designs encompass various types that can be customized to fit specific research objectives and settings. Each design offers unique advantages and limitations, allowing researchers to control for confounding factors, examine interaction effects, or work within ethical constraints. Here are some common types of experimental designs:
Independent Measures
Independent Measures, also known as between-subjects design or independent groups design, is a concept in experimental design where different participants are assigned to different experimental conditions or groups. In this design, each participant experiences only one level of the independent variable, and their responses or outcomes are compared across these different groups.
Using independent measures allows researchers to simultaneously examine multiple conditions, reducing the potential influence of individual differences and participant-related variables. However, it requires a larger sample size and poses the risk of unequal group compositions. To address this, random assignment is often employed to ensure that participants are distributed randomly across the different conditions.
Matched Pairs
In experimental design, researchers have various options to address the challenge of reducing variability and obtaining reliable results. One approach is the use of a matched pairs experimental design, which falls under the category of between-subjects studies. In this design, researchers aim to minimize pre-existing differences between experimental groups by pairing subjects with similar characteristics. Each pair consists of two participants, with one assigned to the treatment group and the other to the control group.
While it may not achieve the same level of matching as within-subjects designs, matched pairs design helps reduce variability between groups and avoids treatment order effects. However, this approach can be time-consuming and is dependent on finding suitable matched pairs. Overall, selecting an appropriate experimental design requires careful consideration of subject area concerns, available resources, and the research question at hand.
Repeated Measures Design
Repeated Measures Design, also known as within-subjects design, is an experimental approach where the same participants are exposed to multiple conditions or levels of an independent variable. Measuring participants’ responses across conditions allows for the examination of within-subject differences while minimizing individual variability. However, it is important to address potential order effects through counterbalancing techniques.
Repeated measures design offers advantages such as increased statistical power and insights into individual variations. Analyzing the data often involves specialized statistical techniques. Overall, repeated measures design provides a valuable method to study changes within participants and understand the effects of independent variables while controlling for individual differences.
Between-subjects Vs. Within-subjects
In a between-subjects design, participants are assigned to different groups, such as a treatment group or a control group, and the groups are compared at the end of the experiment. This approach, also known as independent measures design, ensures that each participant is exposed to only one condition. However, pre-existing differences between groups can potentially impact the results, despite efforts to randomize assignments.
On the other hand, a within-subjects design, also referred to as a repeated measures design, involves participants experiencing all treatment conditions and being measured for each one. This design allows each participant to serve as their control, reducing variability and increasing statistical power. However, the order in which treatments are administered can influence the results, and researchers need to be mindful of potential practice and fatigue effects.
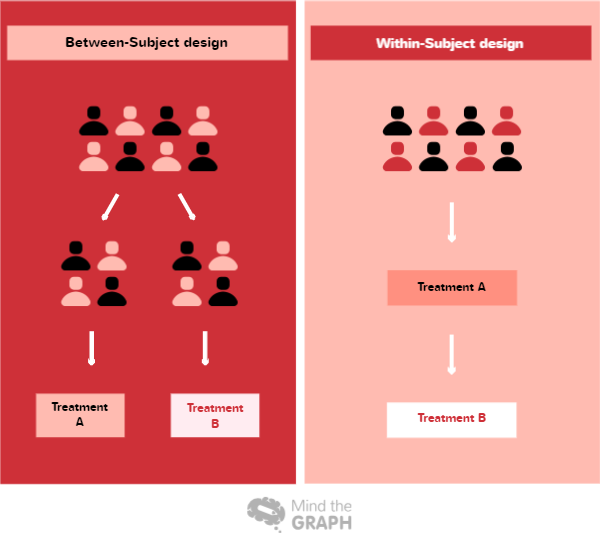
Choosing between a between-subjects design and a within-subjects design requires careful consideration of the research objectives, the nature of the studied variables, and potential confounding factors.
Developing An Experimental Design
Developing an experimental design involves careful planning to optimize the collection of trustworthy data and detect causal relationships. The primary objective of these studies is to observe effects that exist within the population under investigation, with a preference for identifying causal effects. This requires isolating the true effect of each factor from potential confounding variables and generating conclusions that can be generalized to the real world.
To achieve these goals, experimental designs prioritize data validity and reliability and internal and external experimental validity. When an experiment is valid and reliable, researchers can have confidence in the accuracy and consistency of their procedures and data, leading to reliable results.
A successful experimental design involves the following key components:
Preplanning
Significant emphasis is placed on thorough preplanning, where researchers carefully consider the research question, the variables of interest, and the overall design of the experiment. This ensures that all necessary aspects are taken into account before the study begins.
Developing Experimental Treatments
Researchers design and define the treatments or conditions that participants will experience during the experiment. These treatments are carefully designed to manipulate the variables of interest, allowing researchers to assess their effects.
Determining Subject Assignment To Treatment Groups
Researchers must decide how to assign participants or subjects to different treatment groups. This can be done randomly or through other systematic methods to ensure fairness and minimize potential biases.
Assigning Subjects To Experimental Groups
The assignment of subjects to experimental groups is a critical aspect of experimental design. Researchers must carefully determine how participants are allocated to the treatment and control groups. The control group typically represents the absence of treatment and provides a baseline for comparison. The method of assigning subjects to groups significantly impacts the ability to establish true causal effects and control for confounding variables. Let’s examine some approaches to allocating subjects within experimental designs.
Completely Randomized
Completely randomized designs involve randomly assigning subjects to treatment and control groups using methods like flipping coins, rolling dice, or using a computer. This random assignment ensures the groups are roughly equivalent at the beginning, increasing confidence in attributing any differences observed at the end to the treatments rather than other factors. Randomization helps equalize confounders between the groups, leaving only the treatment effects.
An example of a completely randomized design is a study investigating the effects of a new teaching method on student performance. Researchers randomly assign students to two groups: one group receives the new teaching method, while the other group continues with the traditional teaching method. If there are noticeable changes in outcomes by the end of the study, researchers can be sure that the improvements were caused by the new method.
Randomized Block
Randomized block designs are employed when nuisance factors, which can impact outcomes but are not the primary focus of the research, are present. These factors have the potential to conceal or distort treatment effects. To mitigate their influence, experimenters use a randomized block design.
In this design, subjects sharing a common nuisance characteristic are organized into blocks, and participants within each block are randomly assigned to the experimental groups. This approach allows for controlling known nuisance factors. By incorporating blocking in experimental designs, the impact of nuisance variables on experimental error is reduced. The analysis examines treatment effects within each block, removing variability between blocks. Consequently, blocked designs enhance the accuracy of detecting treatment effects by minimizing the influence of nuisance variables .
For instance, if testing different teaching methods, grade level may be a relevant nuisance factor influencing educational outcomes. To implement a randomized block design, participants would be divided by grade level, and members within each level would be randomly assigned to the experimental groups.
Observational Studies
Observational studies, also known as quasi-experimental designs, are utilized in situations where it is impractical or unethical to randomly assign subjects to experimental conditions. Instead, researchers observe subjects within their natural groupings, measure critical variables, and search for correlations.
Observational studies allow for research when treatment control is not feasible. However, quasi-experimental designs introduce challenges related to confounding variables. In this type of experimental design, the correlation between variables does not necessarily indicate causation. While specific procedures can help manage confounders in observational studies, confidence in establishing causal findings is ultimately lower.
For example, imagine you’re studying the impact of exercise on weight loss. It’s not possible to randomly assign participants to exercise and non-exercise groups. However, you can compare individuals who engage in regular exercise with those who don’t and observe how their weight loss outcomes vary.
Treatments In Experimental Designs
In experimental design, treatments refer to the variables that researchers manipulate and control during the study. These treatments serve as the primary independent variables of interest, and the researchers administer them to the subjects or items involved in the experiment. The purpose is to observe whether the treatments have an impact on the outcome or dependent variable.
While treatments can include medical interventions like medications or vaccines, the term extends to various other factors such as training programs, teaching methods, manufacturing settings, or types of fertilizers. When determining the treatments, it is crucial to carefully consider their specific characteristics, such as the dosage or intensity. For example, if comparing three different temperatures in a manufacturing process, it is necessary to define the specific variations between them.
The way treatments are defined and designed within the experiment can significantly impact the results obtained and the generalizability of the findings. Therefore, careful consideration and precise specification of the treatments are important aspects of experimental design to ensure accurate and meaningful conclusions.
Visually Appealing Figures For Your Research
Mind the Graph offers scientists the ability to create visually appealing figures for their research. With a user-friendly interface and an extensive library of scientific illustrations, researchers can easily customize templates, charts, and graphs to convey their findings engagingly. The platform also provides access to scientifically accurate illustrations, allowing researchers to visually represent complex concepts and structures. By enhancing the visual impact of their research through visually appealing figures, scientists can effectively communicate their work and captivate their audience.
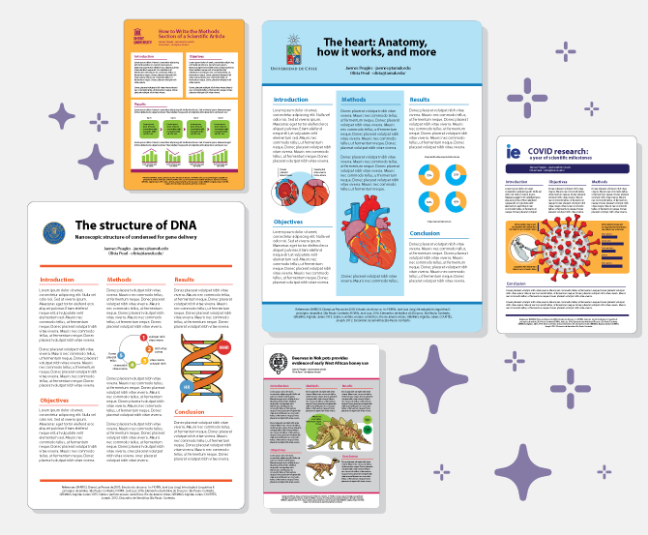
Subscribe to our newsletter
Exclusive high quality content about effective visual communication in science.
Content tags

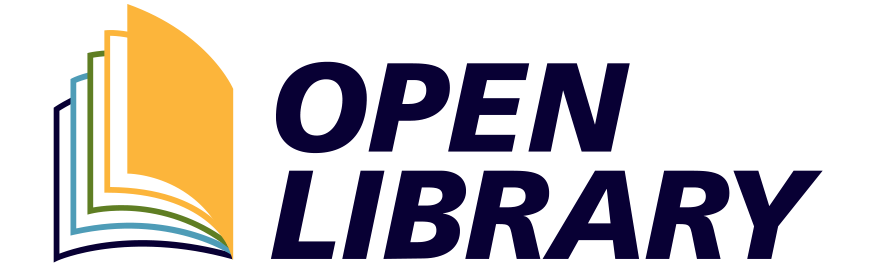
Want to create or adapt books like this? Learn more about how Pressbooks supports open publishing practices.
12.1 Experimental design: What is it and when should it be used?
Learning objectives.
- Define experiment
- Identify the core features of true experimental designs
- Describe the difference between an experimental group and a control group
- Identify and describe the various types of true experimental designs
Experiments are an excellent data collection strategy for social workers wishing to observe the effects of a clinical intervention or social welfare program. Understanding what experiments are and how they are conducted is useful for all social scientists, whether they actually plan to use this methodology or simply aim to understand findings from experimental studies. An experiment is a method of data collection designed to test hypotheses under controlled conditions. Students in my research methods classes often use the term experiment to describe all kinds of research projects, but in social scientific research, the term has a unique meaning and should not be used to describe all research methodologies.
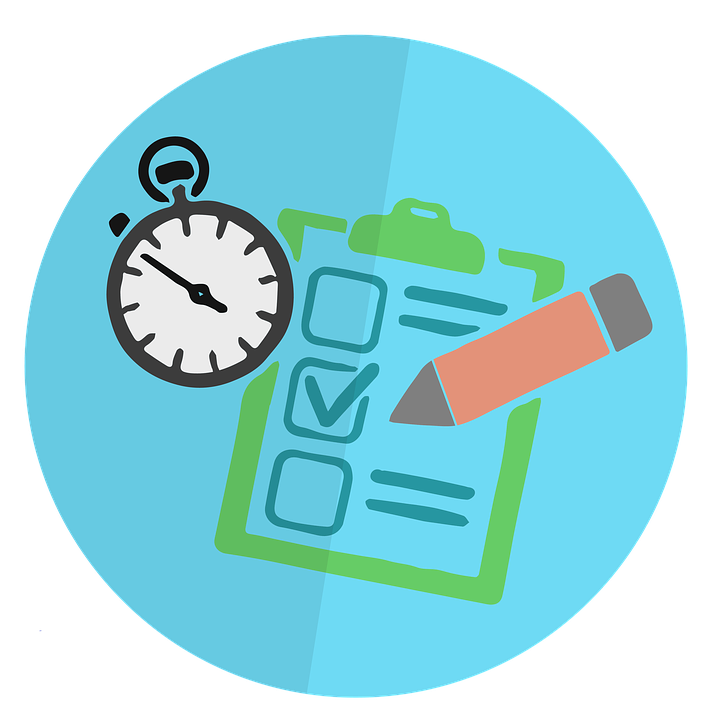
Experiments have a long and important history in social science. Behaviorists such as John Watson, B. F. Skinner, Ivan Pavlov, and Albert Bandura used experimental design to demonstrate the various types of conditioning. Using strictly controlled environments, behaviorists were able to isolate a single stimulus as the cause of measurable differences in behavior or physiological responses. The foundations of social learning theory and behavior modification are found in experimental research projects. Moreover, behaviorist experiments brought psychology and social science away from the abstract world of Freudian analysis and towards empirical inquiry, grounded in real-world observations and objectively-defined variables. Experiments are used at all levels of social work inquiry, including agency-based experiments that test therapeutic interventions and policy experiments that test new programs.
Several kinds of experimental designs exist. In general, designs considered to be true experiments contain three key features: independent and dependent variables, pretesting and posttesting, and experimental and control groups. In a true experiment, the effect of an intervention is tested by comparing two groups: one that is exposed to the intervention (the experimental group , also known as the treatment group) and another that does not receive the intervention (the control group ).
In some cases, it may be immoral to withhold treatment from a control group within an experiment. If you recruited two groups of people with severe addiction and only provided treatment to one group, the other group would likely suffer. For these cases, researchers use a comparison group that receives “treatment as usual.” Experimenters must clearly define what treatment as usual means. For example, a standard treatment in substance abuse recovery is attending Alcoholics Anonymous or Narcotics Anonymous meetings. A substance abuse researcher conducting an experiment may use twelve-step programs in their comparison group and use their experimental intervention in the experimental group. The results would show whether the experimental intervention worked better than normal treatment, which is useful information. However, using a comparison group is a deviation from true experimental design and is more associated with quasi-experimental designs.
Importantly, participants in a true experiment need to be randomly assigned to either the control or experimental groups. Random assignment uses a random number generator or some other random process to assign people into experimental and control groups. Random assignment is important in experimental research because it helps to ensure that the experimental group and control group are comparable and that any differences between the experimental and control groups are due to random chance. We will address more of the logic behind random assignment in the next section.
In an experiment, the independent variable is the intervention being tested—for example, a therapeutic technique, prevention program, or access to some service or support. It is less common in of social work research, but social science research may also have a stimulus, rather than an intervention as the independent variable. For example, an electric shock or a reading about death might be used as a stimulus to provoke a response.
The dependent variable is usually the intended effect the researcher wants the intervention to have. If the researcher is testing a new therapy for individuals with binge eating disorder, their dependent variable may be the number of binge eating episodes a participant reports. The researcher likely expects her intervention to decrease the number of binge eating episodes reported by participants. Thus, she must measure the number of episodes that existed prior to the intervention, which is the pretest , and after the intervention, which is the posttest .
Let’s put these concepts in chronological order so we can better understand how an experiment runs from start to finish. Once you’ve collected your sample, you’ll need to randomly assign your participants to the experimental group and control group. You will then give both groups your pretest, which measures your dependent variable, to see what your participants are like before you start your intervention. Next, you will provide your intervention, or independent variable, to your experimental group. Many interventions last a few weeks or months to complete, particularly therapeutic treatments. Finally, you will administer your posttest to both groups to observer any changes in your dependent variable. Together, this is known as the classic experimental design and is the simplest type of true experimental design. All of the designs we review in this section are variations on this approach. Figure 12.1 visually represents these steps.

An interesting example of experimental research can be found in Shannon K. McCoy and Brenda Major’s (2003) [1] study of peoples’ perceptions of prejudice. In one portion of this multifaceted study, all participants were given a pretest to assess their levels of depression. No significant differences in depression were found between the experimental and control groups during the pretest. Participants in the experimental group were then asked to read an article suggesting that prejudice against their own racial group is severe and pervasive, while participants in the control group were asked to read an article suggesting that prejudice against a racial group other than their own is severe and pervasive. Clearly, these were not meant to be interventions or treatments to help depression, but were stimuli designed to elicit changes in people’s depression levels. Upon measuring depression scores during the posttest period, the researchers discovered that those who had received the experimental stimulus (the article citing prejudice against their same racial group) reported greater depression than those in the control group. This is just one of many examples of social scientific experimental research.
In addition to classic experimental design, there are two other ways of designing experiments that are considered to fall within the purview of “true” experiments (Babbie, 2010; Campbell & Stanley, 1963). [2] The posttest-only control group design is almost the same as classic experimental design, except it does not use a pretest. Researchers who use posttest-only designs want to eliminate testing effects , in which a participant’s scores on a measure change because they have already been exposed to it. If you took multiple SAT or ACT practice exams before you took the real one you sent to colleges, you’ve taken advantage of testing effects to get a better score. Considering the previous example on racism and depression, participants who are given a pretest about depression before being exposed to the stimulus would likely assume that the intervention is designed to address depression. That knowledge can cause them to answer differently on the posttest than they otherwise would. Participants are not stupid. They are actively trying to figure out what your study is about.
In theory, as long as the control and experimental groups have been determined randomly and are therefore comparable, no pretest is needed. However, most researchers prefer to use pretests so they may assess change over time within both the experimental and control groups. Researchers wishing to account for testing effects but also gather pretest data can use a Solomon four-group design. In the Solomon four-group design , the researcher uses four groups. Two groups are treated as they would be in a classic experiment—pretest, experimental group intervention, and posttest. The other two groups do not receive the pretest, though one receives the intervention. All groups are given the posttest. Table 12.1 illustrates the features of each of the four groups in the Solomon four-group design. By having one set of experimental and control groups that complete the pretest (Groups 1 and 2) and another set that does not complete the pretest (Groups 3 and 4), researchers using the Solomon four-group design can account for testing effects in their analysis.
Solomon four-group designs are challenging to implement in the real world because they are time- and resource-intensive. Researchers must recruit enough participants to create four groups and implement interventions in two of them. Overall, true experimental designs are sometimes difficult to implement in a real-world practice environment. It may be impossible to withhold treatment from a control group or randomly assign participants in a study. In these cases, pre-experimental and quasi-experimental designs can be used. However, the differences in rigor from true experimental designs leave their conclusions more open to critique.
Key Takeaways
- True experimental designs require random assignment.
- Control groups do not receive an intervention, and experimental groups receive an intervention.
- The basic components of a true experiment include a pretest, posttest, control group, and experimental group.
- Testing effects may cause researchers to use variations on the classic experimental design.
- Classic experimental design- uses random assignment, an experimental and control group, as well as pre- and posttesting
- Comparison group- a group in quasi-experimental designs that receives “treatment as usual” instead of no treatment
- Control group- the group in an experiment that does not receive the intervention
- Experiment- a method of data collection designed to test hypotheses under controlled conditions
- Experimental group- the group in an experiment that receives the intervention
- Posttest- a measurement taken after the intervention
- Posttest-only control group design- a type of experimental design that uses random assignment, and an experimental and control group, but does not use a pretest
- Pretest- a measurement taken prior to the intervention
- Random assignment-using a random process to assign people into experimental and control groups
- Solomon four-group design- uses random assignment, two experimental and two control groups, pretests for half of the groups, and posttests for all
- Testing effects- when a participant’s scores on a measure change because they have already been exposed to it
- True experiments- a group of experimental designs that contain independent and dependent variables, pretesting and post testing, and experimental and control groups
Image attributions
exam scientific experiment by mohamed_hassan CC-0
- McCoy, S. K., & Major, B. (2003). Group identification moderates emotional response to perceived prejudice. Personality and Social Psychology Bulletin , 29, 1005–1017. ↵
- Babbie, E. (2010). The practice of social research (12th ed.). Belmont, CA: Wadsworth; Campbell, D., & Stanley, J. (1963). Experimental and quasi-experimental designs for research . Chicago, IL: Rand McNally. ↵
Scientific Inquiry in Social Work Copyright © 2018 by Matthew DeCarlo is licensed under a Creative Commons Attribution-NonCommercial-ShareAlike 4.0 International License , except where otherwise noted.
Share This Book
Have a language expert improve your writing
Run a free plagiarism check in 10 minutes, automatically generate references for free.
- Knowledge Base
- Methodology
- A Quick Guide to Experimental Design | 5 Steps & Examples
A Quick Guide to Experimental Design | 5 Steps & Examples
Published on 11 April 2022 by Rebecca Bevans . Revised on 5 December 2022.
Experiments are used to study causal relationships . You manipulate one or more independent variables and measure their effect on one or more dependent variables.
Experimental design means creating a set of procedures to systematically test a hypothesis . A good experimental design requires a strong understanding of the system you are studying.
There are five key steps in designing an experiment:
- Consider your variables and how they are related
- Write a specific, testable hypothesis
- Design experimental treatments to manipulate your independent variable
- Assign subjects to groups, either between-subjects or within-subjects
- Plan how you will measure your dependent variable
For valid conclusions, you also need to select a representative sample and control any extraneous variables that might influence your results. If if random assignment of participants to control and treatment groups is impossible, unethical, or highly difficult, consider an observational study instead.
Table of contents
Step 1: define your variables, step 2: write your hypothesis, step 3: design your experimental treatments, step 4: assign your subjects to treatment groups, step 5: measure your dependent variable, frequently asked questions about experimental design.
You should begin with a specific research question . We will work with two research question examples, one from health sciences and one from ecology:
To translate your research question into an experimental hypothesis, you need to define the main variables and make predictions about how they are related.
Start by simply listing the independent and dependent variables .
Then you need to think about possible extraneous and confounding variables and consider how you might control them in your experiment.
Finally, you can put these variables together into a diagram. Use arrows to show the possible relationships between variables and include signs to show the expected direction of the relationships.
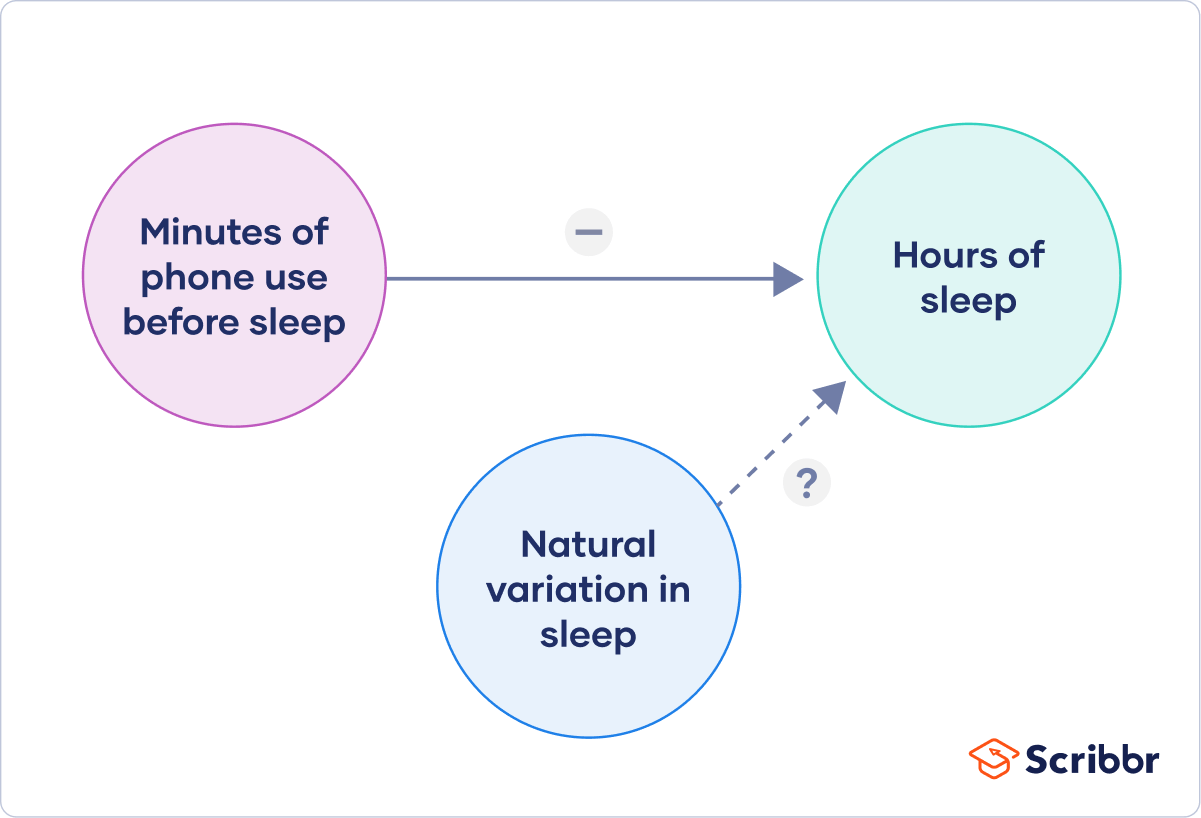
Here we predict that increasing temperature will increase soil respiration and decrease soil moisture, while decreasing soil moisture will lead to decreased soil respiration.
Prevent plagiarism, run a free check.
Now that you have a strong conceptual understanding of the system you are studying, you should be able to write a specific, testable hypothesis that addresses your research question.
The next steps will describe how to design a controlled experiment . In a controlled experiment, you must be able to:
- Systematically and precisely manipulate the independent variable(s).
- Precisely measure the dependent variable(s).
- Control any potential confounding variables.
If your study system doesn’t match these criteria, there are other types of research you can use to answer your research question.
How you manipulate the independent variable can affect the experiment’s external validity – that is, the extent to which the results can be generalised and applied to the broader world.
First, you may need to decide how widely to vary your independent variable.
- just slightly above the natural range for your study region.
- over a wider range of temperatures to mimic future warming.
- over an extreme range that is beyond any possible natural variation.
Second, you may need to choose how finely to vary your independent variable. Sometimes this choice is made for you by your experimental system, but often you will need to decide, and this will affect how much you can infer from your results.
- a categorical variable : either as binary (yes/no) or as levels of a factor (no phone use, low phone use, high phone use).
- a continuous variable (minutes of phone use measured every night).
How you apply your experimental treatments to your test subjects is crucial for obtaining valid and reliable results.
First, you need to consider the study size : how many individuals will be included in the experiment? In general, the more subjects you include, the greater your experiment’s statistical power , which determines how much confidence you can have in your results.
Then you need to randomly assign your subjects to treatment groups . Each group receives a different level of the treatment (e.g. no phone use, low phone use, high phone use).
You should also include a control group , which receives no treatment. The control group tells us what would have happened to your test subjects without any experimental intervention.
When assigning your subjects to groups, there are two main choices you need to make:
- A completely randomised design vs a randomised block design .
- A between-subjects design vs a within-subjects design .
Randomisation
An experiment can be completely randomised or randomised within blocks (aka strata):
- In a completely randomised design , every subject is assigned to a treatment group at random.
- In a randomised block design (aka stratified random design), subjects are first grouped according to a characteristic they share, and then randomly assigned to treatments within those groups.
Sometimes randomisation isn’t practical or ethical , so researchers create partially-random or even non-random designs. An experimental design where treatments aren’t randomly assigned is called a quasi-experimental design .
Between-subjects vs within-subjects
In a between-subjects design (also known as an independent measures design or classic ANOVA design), individuals receive only one of the possible levels of an experimental treatment.
In medical or social research, you might also use matched pairs within your between-subjects design to make sure that each treatment group contains the same variety of test subjects in the same proportions.
In a within-subjects design (also known as a repeated measures design), every individual receives each of the experimental treatments consecutively, and their responses to each treatment are measured.
Within-subjects or repeated measures can also refer to an experimental design where an effect emerges over time, and individual responses are measured over time in order to measure this effect as it emerges.
Counterbalancing (randomising or reversing the order of treatments among subjects) is often used in within-subjects designs to ensure that the order of treatment application doesn’t influence the results of the experiment.
Finally, you need to decide how you’ll collect data on your dependent variable outcomes. You should aim for reliable and valid measurements that minimise bias or error.
Some variables, like temperature, can be objectively measured with scientific instruments. Others may need to be operationalised to turn them into measurable observations.
- Ask participants to record what time they go to sleep and get up each day.
- Ask participants to wear a sleep tracker.
How precisely you measure your dependent variable also affects the kinds of statistical analysis you can use on your data.
Experiments are always context-dependent, and a good experimental design will take into account all of the unique considerations of your study system to produce information that is both valid and relevant to your research question.
Experimental designs are a set of procedures that you plan in order to examine the relationship between variables that interest you.
To design a successful experiment, first identify:
- A testable hypothesis
- One or more independent variables that you will manipulate
- One or more dependent variables that you will measure
When designing the experiment, first decide:
- How your variable(s) will be manipulated
- How you will control for any potential confounding or lurking variables
- How many subjects you will include
- How you will assign treatments to your subjects
The key difference between observational studies and experiments is that, done correctly, an observational study will never influence the responses or behaviours of participants. Experimental designs will have a treatment condition applied to at least a portion of participants.
A confounding variable , also called a confounder or confounding factor, is a third variable in a study examining a potential cause-and-effect relationship.
A confounding variable is related to both the supposed cause and the supposed effect of the study. It can be difficult to separate the true effect of the independent variable from the effect of the confounding variable.
In your research design , it’s important to identify potential confounding variables and plan how you will reduce their impact.
In a between-subjects design , every participant experiences only one condition, and researchers assess group differences between participants in various conditions.
In a within-subjects design , each participant experiences all conditions, and researchers test the same participants repeatedly for differences between conditions.
The word ‘between’ means that you’re comparing different conditions between groups, while the word ‘within’ means you’re comparing different conditions within the same group.
Cite this Scribbr article
If you want to cite this source, you can copy and paste the citation or click the ‘Cite this Scribbr article’ button to automatically add the citation to our free Reference Generator.
Bevans, R. (2022, December 05). A Quick Guide to Experimental Design | 5 Steps & Examples. Scribbr. Retrieved 23 December 2024, from https://www.scribbr.co.uk/research-methods/guide-to-experimental-design/
Is this article helpful?
Rebecca Bevans

IMAGES
COMMENTS
Jul 31, 2023 · Three types of experimental designs are commonly used: 1. Independent Measures. Independent measures design, also known as between-groups, is an experimental design where different participants are used in each condition of the independent variable. This means that each condition of the experiment includes a different group of participants.
The design of experiments, also known as experiment design or experimental design, is the design of any task that aims to describe and explain the variation of information under conditions that are hypothesized to reflect the variation.
May 28, 2024 · Statistical Experimental Design. Statistical experimental design, also known as design of experiments (DOE), is a branch of statistics that focuses on planning, conducting, analyzing, and interpreting controlled tests to evaluate the factors that may influence a particular outcome or process.
These observational studies are also known as quasi-experimental designs. Retrospective studies must be observational in nature because they look back at past events. Imagine you’re studying the effects of depression on an activity. Clearly, you can’t randomly assign participants to the depression and control groups.
Jun 9, 2024 · A within-participant design, also known as a repeated-measures design, is a type of experimental design where the same participants are assigned to multiple groups or conditions. Some advantages of this design are:
Mar 26, 2024 · Types of Experimental Designs. Experimental designs can vary based on the number of variables, the assignment of participants, and the purpose of the experiment. Here are some common types: 1. Pre-Experimental Designs. These designs are exploratory and lack random assignment, often used when strict control is not feasible.
Dec 3, 2019 · An experimental design where treatments aren’t randomly assigned is called a quasi-experimental design. Between-subjects vs. within-subjects. In a between-subjects design (also known as an independent measures design or classic ANOVA design), individuals receive only one of the possible levels of an experimental treatment.
Nov 3, 2023 · Repeated Measures Design, also known as within-subjects design, is an experimental approach where the same participants are exposed to multiple conditions or levels of an independent variable. Measuring participants’ responses across conditions allows for the examination of within-subject differences while minimizing individual variability.
Together, this is known as the classic experimental design and is the simplest type of true experimental design. All of the designs we review in this section are variations on this approach. Figure 12.1 visually represents these steps. Figure 12.1 Steps in classic experimental design
Apr 11, 2022 · An experimental design where treatments aren’t randomly assigned is called a quasi-experimental design. Between-subjects vs within-subjects. In a between-subjects design (also known as an independent measures design or classic ANOVA design), individuals receive only one of the possible levels of an experimental treatment.