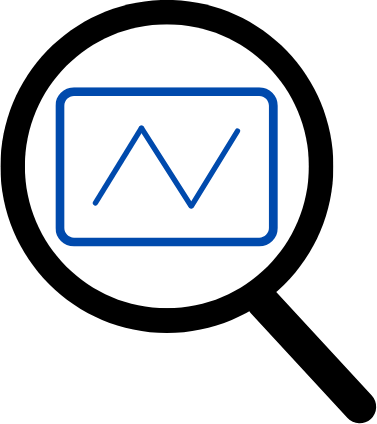
Presentations made painless
- Get Premium

120 Data Management Essay Topic Ideas & Examples
Inside This Article
Data Management Essay Topic Ideas & Examples
Data management is a crucial aspect of any organization's operations. It involves the collection, organization, analysis, and utilization of data to make informed decisions and drive business growth. If you are a student studying data management, you may be required to write essays on various topics related to this field. To help you get started, here are 120 data management essay topic ideas and examples:
- The importance of data management in the digital age.
- Strategies for effective data management in the healthcare industry.
- Ethical considerations in data management and privacy.
- The role of data management in improving customer experience.
- Challenges faced by organizations in managing big data.
- The impact of data management on decision-making processes.
- How data management contributes to business intelligence.
- Data governance: Best practices and its significance.
- The role of data management in ensuring data quality.
- Data management and its impact on risk management.
- The role of data management in improving organizational productivity.
- Data management in the era of IoT (Internet of Things).
- Data management practices in the retail industry.
- The benefits of data management for supply chain optimization.
- Data management in the banking and finance sector.
- The role of data management in improving cybersecurity.
- Challenges and opportunities of data management in the education sector.
- Data management strategies for effective marketing campaigns.
- The impact of data management on improving project management.
- Data management in the transportation and logistics industry.
- Data management and its role in improving sustainability practices.
- The impact of data management on fraud detection and prevention.
- Data management challenges in the entertainment industry.
- The role of data management in disaster management and recovery.
- Data management practices for effective talent management.
- The impact of data management on improving customer relationship management.
- Data management in the hospitality and tourism industry.
- The role of data management in optimizing manufacturing processes.
- Data management practices for effective supply chain visibility.
- The impact of data management on improving product development.
- Data management and its role in improving customer retention.
- Data management challenges in the e-commerce industry.
- The role of data management in enhancing competitiveness.
- Data management practices in the agricultural sector.
- The impact of data management on improving inventory management.
- Data management in the energy and utilities sector.
- The role of data management in optimizing human resource management.
- Data management practices for effective project portfolio management.
- The impact of data management on improving service quality.
- Data management and its role in improving organizational agility.
- Data management challenges in the non-profit sector.
- The role of data management in enhancing government services.
- Data management practices for effective knowledge management.
- The impact of data management on improving sales performance.
- Data management in the real estate industry.
- The role of data management in optimizing fleet management.
- Data management challenges in the pharmaceutical industry.
- The impact of data management on improving customer loyalty.
- Data management practices for effective product lifecycle management.
- The role of data management in optimizing project risk management.
- Data management in the telecommunications sector.
- The impact of data management on improving organizational efficiency.
- Data management and its role in optimizing business process management.
- Data management challenges in the public sector.
- The role of data management in enhancing data-driven decision-making.
- Data management practices for effective customer segmentation.
- The impact of data management on improving employee engagement.
- Data management in the automotive industry.
- The role of data management in optimizing fleet tracking.
- Data management challenges in the manufacturing sector.
- The impact of data management on improving service delivery.
- Data management practices for effective risk assessment.
- The role of data management in optimizing customer satisfaction.
- Data management in the construction industry.
- The impact of data management on improving organizational innovation.
- Data management and its role in optimizing business continuity planning.
- Data management challenges in the retail banking sector.
- The role of data management in enhancing data governance.
- Data management practices for effective market research.
- The impact of data management on improving employee performance.
- Data management in the fashion and apparel industry.
- The role of data management in optimizing customer churn prediction.
- Data management challenges in the healthcare insurance sector.
- The impact of data management on improving business resilience.
- Data management practices for effective social media analytics.
- The role of data management in optimizing project stakeholder management.
- Data management in the food and beverage industry.
- The impact of data management on improving organizational culture.
- Data management and its role in optimizing business intelligence reporting.
- Data management challenges in the hospitality sector.
- The role of data management in enhancing data integration.
- Data management practices for effective customer relationship analytics.
- The impact of data management on improving customer satisfaction.
- Data management in the technology industry.
- The role of data management in optimizing fleet maintenance.
- Data management challenges in the insurance sector.
- The impact of data management on improving business performance.
- Data management practices for effective sentiment analysis.
- The role of data management in optimizing project resource allocation.
- Data management in the pharmaceutical industry.
- The impact of data management on improving organizational agility.
- Data management and its role in optimizing business process reengineering.
- Data management challenges in the oil and gas sector.
- The role of data management in enhancing data privacy.
- The impact of data management on improving employee productivity.
- Data management in the telecommunications industry.
- The role of data management in optimizing fleet optimization.
- Data management challenges in the retail sector.
- The role of data management in optimizing customer loyalty.
These topics cover a wide range of industries and aspects related to data management. Choose a topic that aligns with your interests and expertise, and conduct thorough research before writing your essay. Remember to cite credible sources and provide examples to support your arguments. Good luck with your essay!
Want to research companies faster?
Instantly access industry insights
Let PitchGrade do this for me
Leverage powerful AI research capabilities
We will create your text and designs for you. Sit back and relax while we do the work.
Explore More Content
- Privacy Policy
- Terms of Service
© 2024 Pitchgrade
tableau.com is not available in your region.
An official website of the United States government
Official websites use .gov A .gov website belongs to an official government organization in the United States.
Secure .gov websites use HTTPS A lock ( Lock Locked padlock icon ) or https:// means you've safely connected to the .gov website. Share sensitive information only on official, secure websites.
- Publications
- Account settings
- Advanced Search
- Journal List

Essentials of Data Management: An Overview
Miren b dhudasia , mbbs, mph, robert w grundmeier , md, sagori mukhopadhyay , md, mmsc.
- Author information
- Article notes
- Copyright and License information
Corresponding author: Sagori Mukhopadhyay, MD, MMSc. Roberts Center for Pediatric Research, Children’s Hospital of Philadelphia, 2716 South Street, Office 19-322, Philadelphia, PA 19146. [email protected] . Phone: (215) 829-3603. Fax: (215) 829-7211.
Issue date 2023 Jan.
Users may view, print, copy, and download text and data-mine the content in such documents, for the purposes of academic research, subject always to the full Conditions of use: http://www.nature.com/authors/editorial_policies/license.html#terms
What is data management?
Data management is a multi-step process that involves obtaining, cleaning, and storing data to allow accurate analysis and produce meaningful results. While data management has broad applications (and meaning) across many fields and industries, in clinical research the term data management is frequently used in context of clinical trials. 1 This editorial is written to introduce early career researchers to practices of data management more generally, as applied to all types of clinical research studies.
Outlining a data management strategy prior to initiation of a research study plays an essential role in ensuring that both scientific integrity (i.e., data generated can accurately test the hypotheses proposed) and regulatory requirements are met. Data management can be divided into three steps – data collection, data cleaning and transformation, and data storage. These steps are not necessarily chronological and often occur simultaneously. Different aspects of the process may require expertise of different people necessitating a team effort for effective completion of all steps.
1. Data collection
Data source:.
Data collection is a critical first step in the data management process and may be broadly classified as “primary data collection” (collection of data directly from the subjects specifically for the study) and “secondary use of data” (re-purposing data that was collected for some other reason – either for clinical care in the subject’s medical record or for a different research study). While the terms retrospective and prospective data collection are occasionally used, 2 these terms are more applicable to how the data is utilized rather than how it is collected . Data used in a retrospective study is almost always secondary data; data collected as part of a prospective study typically involves primary data collection, but may also involve secondary use of data collected as part of ongoing routine clinical care for study subjects. Primary data collected for a specific study may be categorized as secondary data when used to investigate a new hypothesis, different from the question for which the data was originally collected. Primary data collection has the advantage of being specific to the study question, minimize missingness in key information, and provide opportunity for data correction in real-time. As a result, this type of data is considered more accurate but increases the time and cost of study procedures. Secondary use of data includes data abstracted from medical records, administrative data such as from hospital’s data warehouse or insurance claims, and secondary use of primary data collected for a different research study. Secondary use of data offers access to large amounts of data that is already collected but often requires further cleaning and codification to align the data with the study question.
Data forms:
A case report form (CRF) is a powerful tool for effective data collection. A CRF is a paper or electronic questionnaire designed to record pertinent information from study subjects as outlined in the study protocol. 3 CRFs are always required in primary data collection but can also be useful in secondary use of data to pre-emptively identify, define and if necessary, derive critical variables for the study question. For instance, medical records provide a wide array of information that may not be required or be useful for the study question. A CRF with well-defined variables and parameters helps the chart reviewer focus only on the relevant data, and makes data collection more objective and unbiased, and in addition, optimize patient confidentiality by minimizing the amount of patient information abstracted. Tools like REDCap (Research Electronic Data Capture) provide electronic CRFs and offer some advanced features like setting validation rules to minimize errors during data collection. 4 Designing an effective CRF upfront during the study planning phase helps to streamline the data collection process, and make it more efficient. 3
2. Data cleaning and transformation
Quality checks:.
Data collected may have errors that arise from multiple sources - data manually entered in a CRF may have typographical errors, whereas data obtained from data warehouses or administrative databases may have missing data, implausible values, and non-random misclassification errors. Having a systematic approach to identify and rectify these errors, while maintaining a log of the steps performed in the process, can prevent many roadblocks during analysis.
First, it is important to check for missing data. Missing data is defined as values that are not available and that would be meaningful for analysis if they were observed. 5 Missing data can bias the results of the study depending on how much data is missing and what is the pattern of distribution of missing data in the study cohort. Many methods for handling missing data have been published. Kang et al provide a practical review of methods for handling missing data. 6 If missing data cannot be retrieved and is limited to only a small number of subjects, one approach is to exclude these subjects from the study. Missing data in different variables across many subjects often require more sophisticated approaches to account for the ‘missingness’. These may include creating a category of ‘missing’ (for categorical variables), simple imputation (e.g., substituting missing values in a variable with average of non-missing values in the variable), or multiple imputation (substituting missing values with the most probable value derived from other variables in the dataset). 7
Second, errors in the data can be identified by running a series of data validation checks. Some examples for data validation rules for identifying implausible values are shown in Table . Automated algorithms for detection and correction of implausible values may be available for cleaning specific variables in large datasets (e.g. growth measurements). 8 After identification, data errors can either be corrected, if possible, or can be marked for deletion. Other approaches, similar to those for dealing with missing data, can also be used for managing data errors.
Examples of validation rules that can assist in data quality checks.
Data transformation:
The data collected may not be in the form required for analysis. The process of data transformation includes re-categorization and re-codification of the data which has been collected along with derivation of new variables, to align with the study analytic plan. Examples include categorizing body mass index collected as a continuous variable into under- and over-weight categories, re-coding free text values such as “growth of an organism” or “no growth”, etc. into a binary ‘positive’ or ‘negative’, or deriving new variables such as average weight per year from multiple weight values over time available in the dataset. Maintaining a code-book of definitions for all variables, pre-defined and derived, can help a data analyst better understand the data.
3. Data storage
Securely storing data is especially important in clinical research as the data may contain protected health information (PHI) of the study subjects. 9 Most institutes that support clinical research have guidelines for safeguards to prevent accidental data breeches.
Data is collected in paper or electronic formats. Paper data should be stored in secure file cabinets inside a locked office at the site approved by the institutional review board. Electronic data should be stored on a secure approved institutional server of the site, and should never be transported using unencrypted portable media devices (e.g. “thumb drives”). If all study team members do not require access to study data, then selective access should be granted to the study team members based on their roles.
Another important aspect of data storage is data de-identification. Data de-identification is a process by which identifying characteristics of the study participants are removed from the data, in order to mitigate privacy risks to individuals. 10 Identifying characteristics of a study subject includes name, medical record number (MRN), date of birth/death, etc. To de-identify data, these characteristics should either be removed from the data or modified (for example, changing MRN to study IDs, changing dates to age/duration, etc.). If feasible, study data should be de-identified when storing. If you anticipate that re-identification of the study participants may be required in future then the data can be separated into two files, one containing only the de-identified data of the study participants, and one containing all the identifying information, with both files containing a common linking variable (e.g., study ID), which is unique for every subject or record in the two files. The linking variable can be used to merge the two files when re-identification is required to carry out additional analyses or to get further data. The link key should be maintained in a secure institutional server accessible only to authorized individuals who need access to the identifiers.
To conclude, effective data management is important to the successful completion of research studies and to ensure the validity of the results. Outlining the steps of the data management process upfront will help streamline the process and reduce the time and effort subsequently required. Assigning team members responsible for specific steps and maintaining a log, with date/time stamp to document each action as it happens, whether you are collecting, cleaning or storing data, can ensure all required steps are done correctly and identify any errors easily. Effective documentation is a regulatory requirement for many clinical trials and is helpful for ensuring all team members are on the same page. When interpreting results, it will serve as an important tool to assess if the interpretations are valid and unbiased. Lastly, it will ensure the reproducibility of the study findings.
Acknowledgments
Statement of financial support: This work was partially supported in part by the Eunice Kennedy Shriver National Institute of Child Health & Human Development of the National Institutes of Health grant (K23HD088753).
Disclosure statement: The authors have no conflicts of interest or financial relationships relevant to this article to disclose.
- 1. Krishnankutty B, Bellary S, Kumar NB, Moodahadu LS. Data management in clinical research: An overview. Indian J Pharmacol. 2012;44(2):168–172. [ DOI ] [ PMC free article ] [ PubMed ] [ Google Scholar ]
- 2. Weinger MB, Slagle J, Jain S, et al. Retrospective data collection and analytical techniques for patient safety studies. J Biomed Inform. 2003;36(1–2):106–119. [ DOI ] [ PubMed ] [ Google Scholar ]
- 3. Avey M Case Report Form Design. In: Rondel RK, Varley SA, Webb CF, eds. Clinical Data Management. 2nd ed. West Sussex, England: John Wiley & Sons Ltd. 1999:47–73. [ Google Scholar ]
- 4. Harris PA, Taylor R, Thielke R, et al. Research electronic data capture (REDCap)—a metadata-driven methodology and workflow process for providing translational research informatics support. J Biomed Inform. 2009;42(2):377–381. [ DOI ] [ PMC free article ] [ PubMed ] [ Google Scholar ]
- 5. Little RJ, D’Agostino R, Cohen ML, et al. The prevention and treatment of missing data in clinical trials. N Engl J Med. 2012;367(14):1355–1360. doi: 10.1056/NEJMsr1203730 [doi]. [ DOI ] [ PMC free article ] [ PubMed ] [ Google Scholar ]
- 6. Kang H The prevention and handling of the missing data. Korean journal of anesthesiology. 2013;64(5):402. [ DOI ] [ PMC free article ] [ PubMed ] [ Google Scholar ]
- 7. Rubin DB. Inference and missing data. Biometrika. 1976;63(3):581–592. [ Google Scholar ]
- 8. Daymont C, Ross ME, Russell Localio A, Fiks AG, Wasserman RC, Grundmeier RW. Automated identification of implausible values in growth data from pediatric electronic health records. Journal of the American Medical Informatics Association. 2017;24(6):1080–1087. [ DOI ] [ PMC free article ] [ PubMed ] [ Google Scholar ]
- 9. Office for Civil Rights, Department of Health and Human Services. Health insurance portability and accountability act (HIPAA) privacy rule and the national instant criminal background check system (NICS). final rule. Fed Regist. 2016;81(3):382–396. [ PubMed ] [ Google Scholar ]
- 10. (OCR), Office for Civil Rights. Methods for de-identification of PHI. https://www.hhs.gov/hipaa/for-professionals/privacy/special-topics/de-identification/index.html . Updated 2012. Accessed Nov 13, 2020.
- View on publisher site
- PDF (47.4 KB)
- Collections
Similar articles
Cited by other articles, links to ncbi databases.
- Download .nbib .nbib
- Format: AMA APA MLA NLM
Add to Collections
Data Integrity and Management Research Paper
Adequate data management in research has become an increasingly important topic nowadays when the modern tools facilitate the gathering of information, and the pure amount of raw data can become overwhelming. Dealing with issues in data management requires accuracy, efficiency and compliance with certain guidelines and accepted standards, including the rules of ethics.
Maintaining the data integrity demands special effort on the part of the researcher. According to the guidelines published by Medicines and Healthcare products Regulatory Agency (MHRA), the universal, suitable for any type of research data, integrity requirements consist of the following points:
Establishing data criticality and inherent integrity risk … [with] overarching data governance system, which should include relevant policies and staff training in the importance of data integrity … the organizational (e.g. procedures) and technical (e.g. computer system access) controls applied to different areas of the quality systems.. [and] designing systems to assure data quality and integrity … in a way that encourages compliance with the principles of data integrity. ( MHRA GMP Data Integrity Definitions and Guidance for Industry , 2015, p. 1-2)
The essential examples of such principles include consistency, legibility, completeness and relevance of the data generated in research, as well as its completeness throughout the time of the research. It is also important to keep in mind the risks, for example, human factor, software and hardware faults, conscious falsification of results, accidental errors, and changes in records or technology, which should be avoided by all means (McDowall, 2013, par. 2-9).
After collecting the data, the researcher may proceed to its interpretation however at that stage it may be difficult to remain unbiased. Focusing on the anticipated results of an experiment can affect the interpretation and even cast discredit on the research in whole. Ted Kaptchuk (2003) describes this effect in his article, claiming that the data-judgment interaction is rarely taken into account as there is no objective way to measure the subjective components of interpretation, while researchers tend to evaluate the evidence supporting their beliefs in a different way than the one that distinctly challenges such convictions (par. 2-3).
However not every research should be considered irrelevant due to the biased interpretation, Robert J. MacCoun (1998) argues that interpretation bias is a common phenomenon with a varied motivational, intentional, and purely cognitive determinants, however there is evidence suggesting that the magnitude of biases is often small and they are subtle; a key role the in identification of biased research interpretation and its sources have played the systematic empirical methods; finally, the biases are not all indefensible and the differing standards of proof are completely acceptable, provided that the researchers are self-conscious about standards and stances, and are explicit about them (p. 36).
Finally, for a researcher there always exists a moral barrier in a form of ethical guidelines. The transfer of research data to fellow researchers or academic institutions should be carried out with great care, and it should be considered whether or not such transfer is necessary.
An advice on data protection and transfer, published at the website of Cardiff University, states that the data should be secured during the transit; sharing the personal data with other organizations should be conducted under the Data Protection Act; access to personal data must be restricted to the minimum number of the research participants, and the issues with identifiability should be avoided as well ( FAQs on Data Protection, n.d., par. 10-20). Another guideline sums it all up: “However simple or complex your data set, think about what you might need to do to ensure that your management of the data respects the terms of your consent, and in particular, the confidentiality and anonymity that participants were promised” ( Data storage and data security , n.d., par. 14).
Reference List
Data storage and data security. (n.d.). Web.
FAQs on Data Protection and Writing a Research Protocol or Applying for Research Ethics Approval. (n.d.). Web.
Kaptchuk, T. J. (2003). Effect of Interpretive Bias on Research Evidence. BMJ: British Medical Journal, 326 (7404), 1453–1455. Web.
MacCoun, R. J. (1998). Biases in the Interpretation and Use of Research Results. Annual Review of Psychology , 49 , 259-287. Web.
McDowall, R. D. (2013). FDA’s Focus on Laboratory Data Integrity – Part 1. Web.
MHRA GMP Data Integrity Definitions and Guidance for Industry . (2015). Web.
- College's Database Purpose and Entities
- Qualitative research: Data Analysis and Reporting Plan
- Popper's Falsification Science
- Resume Falsification: Legal and Moral Responsibility
- In Defense of Marriage Act 1996
- The Trade in Data as Business
- Visual Display of Data: Exploratory Data Analysis
- Information Technology Security Assessment Methodologies
- Regulatory Compliance and Industry Standards
- Data Protection Mechanisms
- Chicago (A-D)
- Chicago (N-B)
IvyPanda. (2022, January 29). Data Integrity and Management. https://ivypanda.com/essays/research-data-management/
"Data Integrity and Management." IvyPanda , 29 Jan. 2022, ivypanda.com/essays/research-data-management/.
IvyPanda . (2022) 'Data Integrity and Management'. 29 January.
IvyPanda . 2022. "Data Integrity and Management." January 29, 2022. https://ivypanda.com/essays/research-data-management/.
1. IvyPanda . "Data Integrity and Management." January 29, 2022. https://ivypanda.com/essays/research-data-management/.
Bibliography
IvyPanda . "Data Integrity and Management." January 29, 2022. https://ivypanda.com/essays/research-data-management/.
- To find inspiration for your paper and overcome writer’s block
- As a source of information (ensure proper referencing)
- As a template for you assignment
- Trending Now
- Foundational Courses
- Data Science
- Practice Problem
- Machine Learning
- System Design
- DevOps Tutorial
What is Data Management?
Over the last few decades, the constant development of cloud systems , artificial intelligence , and the Internet of Things has achieved remarkable growth in collaborating with big data. With the more complex structures, data management contributes a lot at the enterprise level to manage the data. It is most important to understand that the data management system plays an important role in ensuring the smooth functioning of businesses and organizations that mainly deal with data.
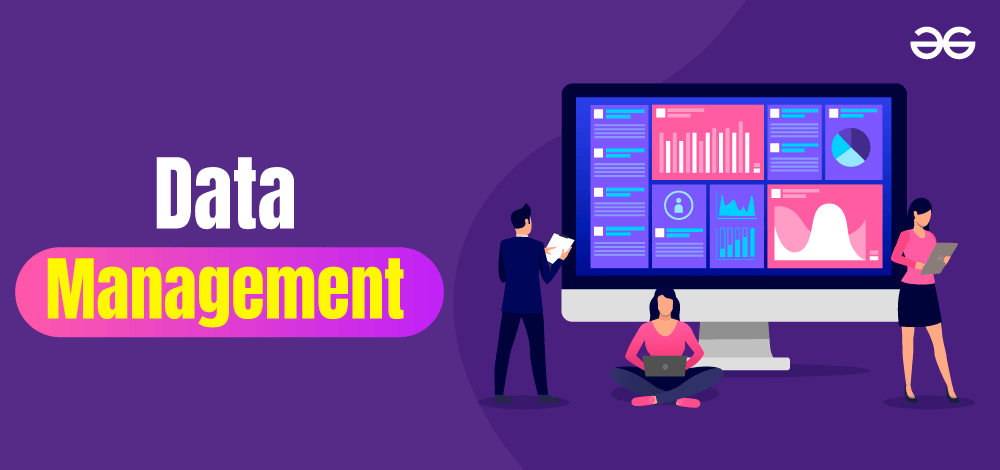
An efficient approach of collecting, filtering, as well as deploying data sets in a structured manner to achieve the projected organization’s goals and decision-making process. In the effective data management system , it is the most important process for the information technology sector. It runs business applications by providing analytical information. The whole process is a combination of different functions that are collectively developed to ensure accessibility to the data. In this blog, we are going to discuss today the critical concepts of data management and its significance, with the associated risks and difficulties of the data handling process.
Data management is a functional system for collecting and analyzing raw datasets. The main goal of this process is to help people, and organizations and optimize the use of data by maintaining the policy and regulations. There are some key components of data management include as:
It is the first and foremost process, where you need to collect and gather the data from various sources in a raw format. They could be structured or unstructured. You need to sort the data in a secure and organized manner. This step involves selecting appropriate storage technology based on the data volume.
Collection of data is the first and foremost process, where you need to collect and gather the data from various sources in a raw format. They could be structured or unstructured. You need to sort the data in a secure and organized manner. This step involves selecting appropriate storage technology based on the data volume. Processing the data is an important process to filter the data into a structured format. It involves data cleaning, aggregating, and enhancing the dataset to make it more meaningful. When you need to assure the accuracy and reliability of the data, you should maintain the data quality which involves the validation rules, and error-checking processes.
Maintaining data security and privacy processes implement security measures to protect the data from unauthorized access, security breaches, and data loss by imposing data encryption and access control. Furthermore, it is important to analyze the data by applying the data mining process , machine learning, and data visualization processes . There are different types of data management lifecycles in the market, by which organizations can maintain the business and regulatory requirements to develop a durable data set. It leads to managing the process of metadata and provides detailed information about the structure , mining process , and data usage to perform the process effectively.
Importance of Data Management
In today’s data-driven world, data management has become a paramount concept, which involves various organizations, storage, processing, and data protection. It increases data accuracy and accessibility to ensure user reliability. Here are some key reasons that make Data Management very important:
- Informed Decision-Making Process: Data is the most important component for businesses and organizations because they make their important decisions based on data. A proper data management process ensures that the decision-makers have direct access to the updated information which helps to make effective choices.
- Data Quality and Efficiency: A well-managed data set leads to a streamlined process, which helps to maintain data quality and efficiency. It reduces error risks and poor decision-making.
- Compliance and Customer Trust: Many organisations have strict regulations to maintain the data management process properly. It also follows effective processes to handle client data responsibly.
- Strategy Development and Innovation: In the modern context, data is a valuable asset that can help organisations to identify trends and potential opportunities with the challenges. It helps to understand the organisations to culture the market trends with customer behaviour. On the other hand, effective data management allows you to analyse the previous data to identify the patterns which lead to the development of new products and solutions.
- Long-term Sustainability: Proper data management helps organisations to plan for the long run. It helps to master data management efficiently by reducing redundancies, data duplication, and unnecessary storage costs.
- Competitive Advantage: Proper data management entitles organisations to explore market trends, customer behaviours, and other insights that can help them outperform competitors.
Data Management Responsibilities and Roles in IT industry
In the IT field, data management involves various job roles and responsibilities to process data properly. Different data management job roles collaborate with the users to handle the different aspects of data management. Here are some common data management jobs in the IT industry –
- Data Manager: They are responsible for overseeing the whole data management strategy. They define the data handling policies and standards by ensuring the data quality, accuracy, and compliance regulations.
- Database Administrator: The job role is related to the database management system . Here the main work is to manage and maintain the databases to store the relevant data by ensuring overall performance and security.
- Data Architect: Data architects design the structure and architecture of the database and whole data systems. It includes the data models and schemas with the developed relationships between the data set to achieve the business requirements.
- Data Analyst: Respective persons perform the data analysis process with data visualisation by analysing the current trends and patterns.
- Data Scientist: They utilise statistical data processing, specifically machine learning techniques, and algorithms to solve complex problems. They mostly collaborate with the businesses and technical teams to deploy the production models.
- Data Security Analyst: They are responsible to implement and manage the security measures to protect the data from breaches and unnecessary access. They monitor all the data access by ensuring the security policies in collaboration with the IT and security teams.
- Chief Data Officers: CDOs hold a strategic role in the IT field and check the data-related activities by defining the data management strategies to achieve the business goals and objectives.
Risks and Challenges in Data Management
While effective data management can produce significant benefits, on the other hand, there are so many risks and challenges related to it. Here are some aspects of them:
- Security and Privacy: Unauthorised access to sensitive data by hacking can be a cause of data breaches, which can expose confidential information and may cause financial losses for an organisation.
- Data Quality: Poor data quality and duplicate data lead to stemming errors during data collection, leading to incorrect decision-making. It occupies valuable storage and creates confusion during the analysis process.
- Data Governance: Lack of data ownership and access control can lead to inconsistent data management. This process leads to security risks and compromises the security of data.
- Data Integration Process: Integrating the data from various sources is difficult, as it contains different formats and complex structures. It disrupts the proper decision-making and process of data analysis.
- Data Scaling: Scaling the data management systems is needed to increase the data loads to maintain the performance by overcoming technical challenges.
- Data Lifecycle Management: Organisations need to be transparent in their data retention policies which helps to determine the data processing time and which data needs to be deleted. Data disposal is also needed for security measures to prevent unauthorised access.
- Data Analysis: Analysing complex and various data sets, required to create the advanced analytics tools. For actionable data insight development, it is needed to understand the business context properly with the particular domain knowledge.
As the IT sectors start to acknowledge the importance of data as their valuable asset in the business world. For that reason, data is also acknowledged by the digital startups and disruptors which have been ahead of the competition in this area for quite some time. Effective data management empowers organisations to transform data into a valuable asset in this competitive and dynamic market. In today’s data-driven world, the data management process provides a crucial way for those organisations who are seeking to promote the power of data.
The data management process offers so many benefits to us, including the top-class decision-making process with an exceptional operational efficiency in the first place. But, we have to remember, there are so many significant challenges and risks related to it as we mentioned here. Addressing the above mentioned challenges we have tried to show you various comprehensive approaches, and how we can solve them with modern policies and advanced data analysis.
Also, Read:
- Data Architecture Design and Data Management
- Difference between Data Management and Data Governance
- Overview of Test data Management (TDM)
FAQs: Data Management
1. what are the most common data management challenges you can face.
Common challenges are mostly related to data silos, inadequate data quality, lack of data maintenance, data security, privacy compliance, and keeping up with rapid technologies.
2. What is Master Data Management?
Master Data Management is an approach to managing and maintaining a single, consistent, authoritative source of master data across an organisation, to ensure data consistency and accuracy.
3. What are the main segments of data management?
Data management includes data governance, data quality, data integration, data modelling, data storage, data security, and data lifecycle management.
4. How can data be stored and managed?
Data can be stored in databases, data warehouses, and data lakes primarily. They provide structured and unstructured data storage options, with various management components.
Similar Reads
- What is Data Management? Over the last few decades, the constant development of cloud systems, artificial intelligence, and the Internet of Things has achieved remarkable growth in collaborating with big data. With the more complex structures, data management contributes a lot at the enterprise level to manage the data. It 8 min read
- What is Data Lifecycle Management? Data lifecycle management provides end-to-end visibility and control over the data flowing through systems and processes. It enables harnessing data as an asset at every stage - from planning through active use and eventual retirement. With strong data lifecycle management, organizations can channel 10 min read
- What is Data Mart? A data mart is a specialized subset of a data warehouse focused on a specific functional area or department within an organization. It provides a simplified and targeted view of data, addressing specific reporting and analytical needs. Data marts are smaller in scale and scope, typically holding rel 6 min read
- What is Data Munging? Data is the foundation of present-day decision-making, yet crude data is frequently messy and unstructured. This is where data munging, or data cleaning, becomes an integral factor. In this article, we'll investigate the meaning of data munging, its key stages, and why it is critical in the data exa 9 min read
- What is Data Retention? Data retention refers to the practice of storing and maintaining data, typically in a digital format, for a specific period. This concept involves determining how long different types of data should be preserved and ensuring compliance with legal, regulatory, or organizational requirements. In this 11 min read
- What is Data Lake ? In the fast-paced world of data science, managing and harnessing vast amounts of raw data is crucial for deriving meaningful insights. One technology that has revolutionized this process is the concept of Data Lakes. A Data Lake serves as a centralized repository that can store massive volumes of ra 7 min read
- What is Data Migration ? In the field of data science and technology, data migration has emerged as an important process for businesses and organizations. As we progress into 2024, the volume and complexity of data have exponentially increased, making the process of transferring data from one system to another a crucial yet 5 min read
- Top Data Management tools in 2024 Data management is a critical practice in the digital age, involving the collection, storage, and organization of an organization's data for future analysis and decision-making. In the field of Information Technology (IT), data management plays a vital role in enabling software developers to efficie 11 min read
- What is Data Transformation? Data transformation is a critical step in data analysis process, encompassing the conversion, cleaning, and organizing of data into accessible formats. This process is critical for businesses looking to utilize their data to generate timely business insights, as it ensures that the information is ac 7 min read
- What is "Transparent DBMS"? A transparent Database Management System (DBMS) has been designed to give users seamless access to data while hiding the complexities of data storage, management, and retrieval. Such a system offers various forms of transparency such as location, fragmentation, replication, concurrency and failure t 6 min read
- What is Data Ingestion? The process of gathering, managing, and utilizing data efficiently is important for organizations aiming to thrive in a competitive landscape. Data ingestion plays a foundational step in the data processing pipeline. It involves the seamless importation, transfer, or loading of raw data from diverse 9 min read
- What is Data Engineering? Data engineering forms the backbone of modern data-driven enterprises, encompassing the design, development, and maintenance of crucial systems and infrastructure for managing data throughout its lifecycle. In this article, we will explore key aspects of data engineering, its key features, importanc 9 min read
- What is Operational Data Stores? An Operational Data Store (ODS) is a type of database that collects and combines data from various sources. It provides a unified view of this data and offers real-time or near-real-time updates. Unlike data warehouses that focus on storing historical data, an ODS is designed to handle current opera 11 min read
- What is a Data Science Platform? In the steadily advancing scene of data-driven navigation, associations are progressively going to refine apparatuses and advancements to bridle the force of data. One such essential component in data examination is the Data Science Platform. This article means to demystify the idea, investigate its 14 min read
- What is a Vector Database? In the field of data handling, the standard database has been an icon for storing and retrieving data. Nevertheless, despite the fact that the amount of data and complexity are constantly increasing, there are new technologies appearing that break the previous limitations of conventional database sy 6 min read
- Types of Database Management Systems A Database Management System (DBMS) is a software system that is designed to manage and organize data in a structured manner. It allows users to create, modify, and query a database, as well as manage the security and access controls for that database. What is DBMS?A DBMS (Database Management System 5 min read
- 12 Best Database Management Software in 2024 Database management software is crucial in today's digital age where data serves as a vital asset for businesses. Organizations rely on database management systems (DBMS) to efficiently store, access, and secure their data. A DBMS is essential software that enables users to define, create, maintain, 14 min read
- Data Quality Management - Definition, Importance In this age, Data plays an important role. Every click, every transaction, and every interaction generates some data. But what happens when this information is inaccurate, incomplete, or inconsistent? This is where Data Quality Management (DQM) comes in. Let’s explore Data Quality Management and und 10 min read
- What is Data Center Security? Whether a business, or a customer, data is integral part of any kind of exchange of goods or services. This data can be in many forms. As an example, the way an organization functions, the ideas an organization is working on, and the internal policies of the organization is all a form of data that n 9 min read
Improve your Coding Skills with Practice
What kind of Experience do you want to share?
We use cookies to enhance our website for you. Proceed if you agree to this policy or learn more about it.
- Essay Database >
- Essays Examples >
- Essay Topics
Essays on Data Management
91 samples on this topic
Crafting a lot of Data Management papers is an inherent part of contemporary studying, be it in high-school, college, or university. If you can do that single-handedly, that's just awesome; yet, other learners might not be that fortunate, as Data Management writing can be quite difficult. The collection of free sample Data Management papers presented below was compiled in order to help struggling learners rise up to the challenge.
On the one hand, Data Management essays we publish here precisely demonstrate how a really well-written academic piece of writing should be developed. On the other hand, upon your request and for an affordable price, a pro essay helper with the relevant academic experience can put together a high-quality paper model on Data Management from scratch.
Learn To Craft Research Papers On Telecommuting And Mobile Worker With This Example
Introduction
The world is rapidly changing especially with the increased embrace of internet use. Telecommuting is quickly gaining momentum as many people prefer working from their living rooms. There are various consequences that can be attributed to this trend.
Impacts of telecommuting
Good Research Paper On Database System And Models
In order to get the commission for each employ we first have to generate a database and its tables. CREATE TABLE Employee( EmpNumber int not null, EmpFirstName varchar(100) not null, EmpLastName varchar(100) not null, CommissionRate decimal(3,2) not null, YrlySalary int not null, DepartmentID int, JobID int, PRIMARY KEY (EmpNumber), FOREIGN KEY (DepartmentID) references Department(DepartmentID), FOREIGN KEY (JobID) references Job(JobID) ).
CREATE TABLE Job( JobID int not null, JobDescription varchar(400) not null, PRIMARY KEY (JobID) )
An Institutional Repository: Digital Archiving And Preservation Report Examples
Paper due date: exemplar essay to follow, health care: example question & answer by an expert writer to follow.
Question One
High-Level Description/Purpose Of Information System Essay
Introducing Azure
Expertly Written Essay On Cogent Communications To Follow
Free report about safety management: blue coral copters inc., good example of essay on healthy work environments and nurse burn out.
Healthy Work Environments and Nurse Burn Out (4)
Draw Topic & Writing Ideas From This Thesis Proposal On Big Data For Product And Process Optimization
CHAPTER ONE: INTRODUCTION
Sample Literature Review On A Critical Review Of The Literature Evaluating The Uptake Of
HPV vaccination among adolescent girls in the UK
Global Information Infrastructure (Gii) Critical Thinking Samples
Sample essay on organizational information systems, the importance of quality information governance in health and the effect it has on healthcare research paper samples, applying diffusion principles for adoption of 3d printing: free sample critical thinking to follow.
3D printing takes a representation of an object in a digital file and uses a process that lays consecutive layers of material to develop a tangible three-dimensional replica of the object. This process used in creating the solid object is known as additive manufacturing (Munoz and Guenther, 2014). The 3D printing technology has given rise to a new type of prototyping known as rapid prototyping. Manufacturers have been the biggest gainers of rapid prototyping because the process allows them to quickly create and change prototypes.
3D Printing in Geographic Information Systems (GIS)
Free Benchmark – Research Critique Essay Example
Expertly crafted essay on quantitative research critique.
Trotter, B., Conaway, M. R., & Burns, S. M. (2013). Relationship of glucose values to sliding scale insulin (correctional insulin) dose delivery and meal time in acute care patients with diabetes mellitus. Medsurg Nursing, 22(2), 99.
This research critique demonstrates the aspects of protection of human subjects in the study of the relationship between glucose and insulin.
Thesis statement
Write By Example Of This Data Quality And MIS Infrastructure Essay
[The Course]
Social Media In Epidemiology Research Course Work Samples
The use of social media in epidemiological studies remains an area that is viewed with skepticism. Epidemiologists often track disease trends following tip-offs and concerns gathered from traditional channels of information (Garrity, 2011). In this study, the researcher will use social media as a tool for gathering information about the eating and exercise patterns of the participants. The researcher will track the updates and photos put up by the participants on Face Book.
Article On High Availability Of Database
The digital file development presentation.
I believe that the integration of digital technology in designs is very necessary and cannot be avoided. This is because of its extensive effects on architecture and its general ambiguity. This paper, I believe, will make it possible for individuals and institutions to analyze and decide appropriately on the use of digital prefabrication, as a new technology in the design systems. Although many opinions have been presented with different position statements, the immediacy of proactive consideration on the digital systems is of utmost importance. In this paper, I have explored the relationship between CAD and CAM, computer and production.
Solidworks Software Article Samples
Free operations analysis presentation example.
Operations analysis is a designed business strategy used by organizations to determine whether the overall operational structure adheres to the proposed business plan adopted and utilized by the organization (Tatum and Bronwyn). The supply chain operations form a vital area in any business because it involved the supply and delivery of goods and services offered by a given company. There has been a rapid evolvement in the discipline of supply chain management creating many challenges to the current and future managers who have to move at the same rate the new analytical techniques are moving
WAL-MART BACKGROUND INFORMATION
Dissertation On Healthcare Delivery At Arms-Length
Final assessment exercise essay example, free data standards and security course work example.
Data security is an important aspect when handling data that is crucial and important in an organization. Data formats form a basis for creating secure data management and data processing processes so that the data formats are secured. There are strategies that can be integrated to data management and processing procedures to ensure that there is secure data. One of the strategies is to ensure that there is data encryption every time the data is sent over a public channel.
Example Of Course Work On Im It Governance And Decision Rights
Course work on multivariate technique, course work on big data, cloud computing change course work examples.
1. How will Cloud Computing change the traditional IT infrastructure?

Structured Data Management Course Work Examples
Although the company continues to come up with ways to promote structured data within the organization, this remains an ongoing effort and is not yet a completely solved problem. At most, company data can be said to be semi-structured.
Some of the company’s structured data management efforts include the use of tools for
Course Work On Databases
A database is an organized collection of data in digital form stored in an electronic device. Databases store data in different formats which can be retrieved for use easily and effectively. The organization of data in databases models reality relevant in real life scenario. The manner in which it is modeled is such that it supports all the processes requiring the information. The term databases therefore refer to the logical and physical implementation of structures that support the storage and retrieval of data
Free Data Report Example
Executive Summary
Audit Academic Report Academic Reports Examples
Discussion 1
Good Example Of Big Data Thesis Proposal
Big data and its value to small medium enterprise sme's restaurants essay sample.
Governance of big data:
Essay On Data Collection
Hubble space telescope instrumentation and scientific discoveries research paper example, free research paper on database use.
Database technology has formed an important aspect of data management at Google. There are many requirements that require data storage at Google and are the reason why there is a big data store for the company. It is important for the company to undertake data storage.
Use of database management system
Good Database Administration As An It Career Essay Example
Good legal and regulatory implications of adopting technologies essay example, free case study on enterprise architecture, oracle erp report samples, good essay on critique of relational database technology.
Other Details
Example Of Research Paper On Documentation Analysis
Example of data analysis report, example of reduced labor costs report.
Business Intelligence and enterprise data mining management
Project Management Software Research Project Research Papers Example
Free research paper about fortune 500 company of 2013, global partners, free the federal no child left behind (nclb) education reform law report sample.
Strategic Management
Abstract This paper discusses about the federal no child left behind (NCLB) education reform law. In the second section the paper discusses about the impact of legislation on local school system. In the third part the recommendations or the suggestions are made to make the said Act more successful. The NCLB requires all schools and districts with the states to ensure all students in the grade level math and also in the reading by 2014. It is the state that defines the grade level performance.
Fiction Corporations Network Design Term Paper Samples
Executive summary
Evidence-Based Project Implementation Issues Research Proposal Examples
More often than not, project implementation does not proceed in the exact manner envisioned by the implementer. There are many factors that could positively or negatively influence the project in unexpected ways. Such a scenario also leads to digressions from best practice. Thus, it is important to monitor the implementation, establish the root cause, and proactively manage arising issues to ensure success. During the evaluation, opportunities for improvement should be explored with regard to managing implementation issues.
Issues in Implementation and Data Gathering
Example Of Essay On Short Statement On My Role As Head Of It Management Effort (It Manager) At Magnum
Free term paper on oracle.
American Public University System
Example Of Big Data Essay
BIG DATA and its value to Small Medium Enterprise SME’s Restaurants
Free Research Paper About Data Warehousing
Introduction and definition of data warehouse
Example Of Case Study On Enterprise Architecture
Question 1 Describe a 'future state' that improves upon their architecture.
Example Of Enterprise Architecture Case Study
Good example of transmittal letter essay.
275 words = 1 page double-spaced
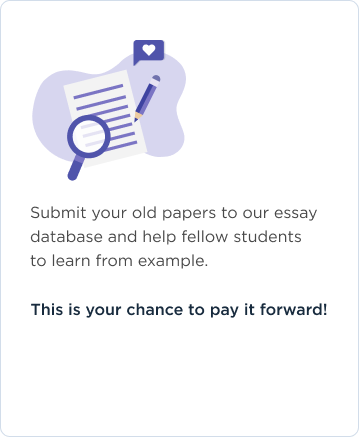
Password recovery email has been sent to [email protected]
Use your new password to log in
You are not register!
By clicking Register, you agree to our Terms of Service and that you have read our Privacy Policy .
Now you can download documents directly to your device!
Check your email! An email with your password has already been sent to you! Now you can download documents directly to your device.
or Use the QR code to Save this Paper to Your Phone
The sample is NOT original!
Short on a deadline?
Don't waste time. Get help with 11% off using code - GETWOWED
No, thanks! I'm fine with missing my deadline
What is data management?
3 july 2024, jim holdsworth.
Data management is the practice of collecting, processing and using data securely and efficiently for better business outcomes.
72% of top-performing CEOs agree that competitive advantage depends on who has the most advanced generative AI . However, in order to take advantage of artificial intelligence (AI), organizations must first organize their information architecture to make their data accessible and usable. Fundamental data management challenges include data volumes, and data silos across multiple locations and cloud providers. New data types and various formats such as documents, images and videos, also present challenges. Also, complexity and inconsistent datasets can limit an organization’s ability to use data for AI.
As a result of these challenges, an effective data management strategy has become an increasing priority for organizations to address challenges presented by big data . A flexible, modern data management system integrates with existing technology within an organization to access high-quality, usable data for data scientists, AI and machine learning (ML) engineers, and the organization’s business users.
A complete data management strategy accounts for various factors, including how to:
- Collect, integrate and store data from diverse sources—including structured and unstructured data—and from across hybrid and multiple clouds.
- Maintain high-availability, resiliency and disaster recovery of data stored across multiple locations.
- Build or obtain fit-for-purpose databases to meet a variety of workload and price-performance needs.
- Help ensure sharing of business data and metadata across organizations, to enable greater self-service, collaboration and access to data.
- Secure and govern data, while helping meet compliance requirements and meeting data privacy requirements.
- Manage the data lifecycle from creation to deletion with end-to-end integration, governance, lineage, observability and master data management (MDM) .
- Automate data discovery and analysis with generative AI for data management.

The latest AI News + Insights
Discover expertly curated insights and news on AI, cloud and more in the weekly Think Newsletter.
Why data management is important
While the data management tools for constructing generative AI applications are widely available, the data itself holds the value for both customers and businesses. High volumes of quality data must be properly organized and processed to successfully train models. This approach is a rapidly growing use case for modern data management.
For example, a generative AI-driven commentary was offered during The Championships 2023 at Wimbledon , which accessed information from 130 million documents and 2.7 million pertinent contextual data points in real time. Visitors using the tournament app or website were able to access complete statistics, play-by-play narration and game commentary, as well as a precise prediction of the winner at any moment as matches progressed. Having the correct data management strategy can help ensure that valuable data is always available, integrated, governed, secure and accurate.
Transforming data into a trusted asset
Generative AI can give organizations a strong competitive advantage, with their AI strategy relying on the strength of the data that’s used. Many organizations still struggle with fundamental data challenges that are exacerbated by the demand for generative AI, which requires ever more data—leading to yet more data management headaches.
Data might be stored in multiple locations, applications and clouds, often leading to isolated data silos. To add even more complexity, the uses of data have become more varied, with data in varying and complex forms—such as images, videos, documents and audio. More time is required for data cleaning, integration and preparation. These challenges can lead organizations to avoid using their full data estate for analytics and AI purposes.
However, equipped with modern tools for data architecture , governance and security, data can be successfully used to gain new insights and make more precise predictions consistently. This capability can enable a deeper understanding of customer preferences and can enhance customer experiences (CX) by delivering insights derived from data analysis . Moreover, it facilitates the development of innovative data-driven business models, such as service offerings reliant on generative AI, which need a foundation of high-quality data for model training.
Creating the right data foundation for digital transformation
Data and analytics leaders face major challenges when transforming their organizations due to the increasing complexity of the data landscape across hybrid cloud deployments. Generative AI and AI assistants , machine learning (ML) , advanced analytics, Internet of Things (IoT) , and automation also all require huge volumes of data to work effectively. This data needs to be stored, integrated, governed, transformed and prepared for the right data foundation. And to build a strong data foundation for AI, organizations need to focus on building an open and trusted data foundation, which means creating a data management strategy that is centered on openness, trust and collaboration.
The AI requirement was summed up by a Gartner® analyst 1 : “AI-ready data means that your data must be representative of the use case, including all patterns, errors, outliers and unexpected emergence that is needed to train or run the AI model for the specific use.”
Data and analytics executives might feel that AI-prepared data equals high-quality data, but the standards of high-quality data for purposes other than AI do not necessarily meet the standard for AI readiness. In the realm of analytics, for instance, data is typically refined to eliminate outliers or conform to human expectations. However, when training an algorithm, it needs representative data.
Ensuring governed, compliant and secure data
Data governance is a subset of data management. This means that when a data governance team identifies commonalities across disparate datasets and wants to integrate them, they will need to partner with a database architecture or engineering team to define the data model and data architecture to facilitate linkages and data flows. Another example pertains to data access. A data governance team might set the policies around data access to specific types of data, such as personally identifiable information (PII) . Meanwhile a data management team would either provide direct access or set a mechanism in place to provide access, such as adjusting internally defined user roles to approve access.
Effective data management, including robust data governance practices, can help with adhering to regulatory compliance. This compliance encompasses both national and global data privacy regulations, such as the General Data Protection Regulation (GDPR) and the California Consumer Privacy Act (CCPA) , along with industry-specific privacy and security standards. Establishing comprehensive data management policies and procedures becomes crucial for demonstrating or undergoing audits to validate these protections.
Is data management the secret to generative AI?
Explore why high-quality data is essential for the successful use of generative AI.
Key aspects of data management
Modern data management solutions provide an efficient way to manage data and metadata across diverse datasets. Modern systems are built with the latest data management software and reliable databases or data stores . This can include transactional data lakes , data warehouses or data lakehouses , combined with a data fabric architecture including data ingestion, governance, lineage, observability and master data management. Together, this trusted data foundation can feed quality data to data consumers as data products, business intelligence (BI) and dashboarding, and AI models—both traditional ML and generative AI.
A strong data management strategy typically includes multiple components to streamline strategy and operations throughout an organization.
The right databases and data lakehouse architecture
While data can be stored before or after data processing, the type of data and purpose of it will usually dictate the storage repository that is used. While relational databases organize data into a tabular format, nonrelational databases do not have as rigid of a database schema.
Relational databases are also typically associated with transactional databases , which run commands or transactions collectively. An example is a bank transfer. A defined amount is withdrawn from one account and then it is deposited within another. But for enterprises to support both structured and unstructured data types, they require purpose-built databases. These databases must also cater to various use cases across analytics, AI and applications. They must span both relational and nonrelational databases, such as key-value, document, wide-column, graph and in-memory . These multimodal databases provide native support for different types of data and the latest development models, and can run many kinds of workloads, including IoT, analytics, ML and AI.
Data management best practices suggest that data warehousing be optimized for high-performance analytics on structured data. This requires a defined schema to meet specific data analytics requirements for specific use cases, such as dashboards, data visualization and other business intelligence tasks. These data requirements are usually directed and documented by business users in partnership with data engineers, who will ultimately run against the defined data model.
The underlying structure of a data warehouse is typically organized as a relational system that uses a structured data format, sourcing data from transactional databases. However, for unstructured and semistructured data, data lakes incorporate data from both relational and nonrelational systems, and other business intelligence tasks. Data lakes are often preferred to the other storage options because they are normally a low-cost storage environment, which can house petabytes of raw data.
Data lakes benefit data scientists in particular, as they enable them to incorporate both structured and unstructured data into their data science projects. However, data warehouses and data lakes have their own limitations. Proprietary data formats and high storage costs limit AI and ML model collaboration and deployments within a data warehouse environment.
In contrast, data lakes are challenged with extracting insights directly in a governed and performant manner. An open data lakehouse addresses these limitations by handling multiple open formats over cloud object storage and combines data from multiple sources, including existing repositories, to ultimately enable analytics and AI at scale.
Hybrid cloud database strategy
Multicloud and hybrid strategies are steadily becoming more popular. AI technologies are powered by massive amounts of data that require modern data stores that reside on cloud-native architectures to provide scalability, cost optimization, enhanced performance and business continuity. According to Gartner 2 , by the end of 2026, "90% of data management tools and platforms that fail to support multi-cloud and hybrid capabilities will be set for decommissioning."
While existing tools aid database administrators (DBAs) in automating numerous conventional management duties, manual involvement remains necessary due to the typically large and intricate nature of database setups. Whenever manual intervention becomes necessary, the likelihood of errors rises. Minimizing the necessity for manual data management stands as a primary goal in operating databases as fully managed services.
Fully managed cloud databases automate time-consuming tasks such as upgrades, backups, patching and maintenance. This approach helps free DBAs from time-consuming manual tasks to spend more time on valuable tasks such as schema optimization, new cloud-native apps and support for new AI use cases. Unlike on-premises deployments, cloud storage providers also enable users to spin up large clusters as needed, often requiring only payment for the storage specified. This means that if an organization needs more compute power to run a job in a few hours (versus a few days), it can do this on a cloud platform by purchasing more compute nodes.
This shift to cloud data platforms is also facilitating the adoption of streaming data processing. Tools such as Apache Kafka enable more real-time data processing, so that consumers can subscribe to topics to receive data in a matter of seconds. However, batch processing still has its advantages as it’s more efficient at processing large volumes of data. While batch processing abides by a set schedule, such as daily, weekly or monthly, it is ideal for business performance dashboards, which typically do not require real-time data.
Data fabric architecture
More recently, data fabrics have emerged to assist with the complexity of managing these data systems. Data fabrics use intelligent and automated systems to facilitate end-to-end integration of data pipelines and cloud environments. A data fabric also simplifies delivery of quality data and provides a framework for enforcing data governance policies to help ensure that the data used is compliant. This facilitates self-service access to trustworthy data products by connecting to data residing across organizational silos, so that business leaders gain a more holistic view of business performance. The unification of data across HR, marketing, sales, supply chain and others give leaders a better understanding of their customer. A data mesh might also be useful. A data fabric is an architecture that facilitates the end-to-end integration. In contrast, a data mesh is a decentralized data architecture that organizes data by a specific business domain—for example, marketing, sales, customer service and more. This approach provides more ownership to the producers of a dataset.
Data integration and processing
Within this stage of the data management lifecycle , raw data is ingested from a range of data sources, such as web APIs , mobile apps , Internet of Things (IoT) devices, forms, surveys and more. After data collection, the data is usually processed or loaded by using data integration techniques, such as extract, transform, load (ETL) or extract, load, transform (ELT) . While ETL has historically been the standard method to integrate and organize data across different datasets, ELT has been growing in popularity with the emergence of cloud data platforms and the increasing demand for real-time data.
In addition to batch processing, data replication is an alternative method of integrating data and consists of synchronizing data from a source location to one or more target locations, helping ensure data availability, reliability and resilience. Technology such as change data capture (CDC) uses log-based replication to capture changes made to data at the source and propagate those changes to target systems, helping organizations make decisions based on current information.
Independently of the data integration technique used, the data is usually filtered, merged or aggregated during the data processing stage to meet the requirements for its intended purpose. These applications can range from a business intelligence dashboard to a predictive machine learning algorithm.
Using continuous integration and continuous deployment (CI/CD) for version control can enable data teams to track changes to their code and data assets. Version control enables data teams to collaborate more effectively, as they can work on different parts of a project simultaneously and merge their changes without conflicts.
Data governance and metadata management
Data governance promotes the availability and usage of data. To help ensure compliance, governance generally includes processes, policies and tools around data quality , data access, usability and data security. For instance, data governance councils tend to align taxonomies to help ensure that metadata is added consistently across various data sources. A taxonomy can also be further documented through a data catalog to make the data more accessible to users, facilitating data democratization across an organization.
Enriching data with the right business context is critical for the automated enforcement of data governance policies and data quality. This is where service level agreement (SLA) rules come into effect, helping ensure that data is protected and of the required quality. It is also important to understand the provenance of the data and gain transparency into the journey of the data as it moves through pipelines. This calls for robust data lineage capabilities to drive visibility as organizational data makes it ways from data sources to the end users. Data governance teams also define roles and responsibilities to help ensure that data access is provided appropriately. This controlled access is particularly important to maintain data privacy.
Data security
Data security sets guardrails in place to protect digital information from unauthorized access, corruption or theft. As digital technology becomes an increasing part of our lives, more scrutiny is placed upon the security practices of modern businesses. This scrutiny is important to help protect customer data from cybercriminals or to help prevent incidents that need disaster recovery. While data loss can be devastating to any business, data breaches, in particular, can result in costly consequences from both a financial and brand standpoint. Data security teams can better secure their data by using encryption and data masking within their data security strategy.
Data observability
Data observability refers to the practice of monitoring, managing and maintaining data in a way that helps ensure its quality, availability and reliability across various processes, systems and pipelines within an organization. Data observability is about truly understanding the health of an organization’s data and its state across a data ecosystem. It includes various activities that go beyond traditional monitoring, which only describes a problem. Data observability can help identify, troubleshoot and resolve data issues in near-real time.
Master data management
Master data management (MDM) focuses on the creation of a single, high-quality view of core business entities including products, customers, employees and suppliers. By delivering accurate views of master data and their relationships, MDM enables faster insights, improved data quality and compliance readiness. With a single 360-degree view of master data across the enterprise, MDM enables businesses with the right data to drive business analytics, determine their most successful products and markets, and their highest valued customers.
Benefits of data management
Organizations experience multiple benefits when starting and maintaining data management initiatives.
Reduced data silos
Many companies inadvertently create data silos within their organization. Modern data management tools and frameworks, such as data fabrics and data lakes, help to eliminate data silos and dependencies on data owners. For instance, data fabrics assist in revealing potential integrations across disparate datasets across functions, such as human resources, marketing and sales. However, data lakes ingest raw data from those same functions, removing dependencies and eliminating single owners to a dataset.
Improved compliance and security
Governance councils assist in placing guardrails to protect businesses from fines and negative publicity that can occur due to noncompliance to government regulations and policies. Missteps here can be costly from both a brand and financial perspective.
Enhanced customer experience
While this benefit might not be immediately seen, successful proof of concepts can improve the overall user experience , enabling teams to better understand and personalize the customer journey through more holistic analyses.
Scalability
Data management can help businesses scale, but this largely depends on the technology and processes in place. For example, cloud platforms enable greater flexibility, so that data owners can scale up or scale down their compute power as needed.
New data management components
Over the last decade, developments within hybrid cloud, artificial intelligence, the Internet of Things (IoT) and edge computing have led to the exponential growth of big data, creating even more complexity for enterprises to manage. New components continue to improve data management capabilities. Here are some of the latest:
Augmented data management
To further boost data management capabilities, augmented data management is becoming increasingly popular. This is a branch of augmented intelligence, powered by cognitive technologies, which include AI, ML, data automation, data fabric and data mesh. The benefits of this automation include enabling data owners to create data products such as catalogs of data assets, with the ability to search and find data products, and query visuals and data products by using APIs. In addition, insights from data fabric metadata can help automate tasks by learning from patterns as part of the data product creation process or as part of the data management process of monitoring data products.
Generative AI
A data store for generative AI such as IBM® watsonx.data™ can help organizations unify, curate and prepare data efficiently for AI models and applications. Integrated and vectorized embedding capabilities enable retrieval-augmented generation (RAG) use cases at scale across large sets of trusted, governed data.
Hybrid cloud deployments
To simplify application connectivity and security across platforms, clusters and clouds, a hybrid cloud deployment can assist. Applications can be easily deployed and moved between environments because containers and object storage have made computing and data portable.
Semantic layer
To accelerate data access and unlock new data insights without SQL, organizations are creating an embeddable, AI-powered semantic layer . This is a metadata and abstraction layer that is built onto the organization’s source data, such as a data lake or warehouse. The metadata can enrich the data model being used and also be sufficiently clear for business users to understand.
Shared metadata layer
Organizations can access data across a hybrid cloud by connecting storage and analytics environments. This access can be through a single point of entry with a shared metadata layer across clouds and on-premises environments. Multiple query engines can be used to optimize analytics and AI workloads.
Creating a shared metadata layer in a data lakehouse to catalog and share data is a best practice. This speeds discovery and enrichment, the analysis of data across multiple sources, the running of multiple workloads and use cases.
In addition, a shared metadata management tool speeds the management of objects in a shared repository. It can be used to add a new host system, add a new database or data file, or add a new schema, in addition to deleting items from a shared repository.
1 Wire19.com: “ Ways to ensure that your data is AI-ready ”, 14 June 2024 2 Gartner: " Strategic Roadmap for Migrating Data Management Solutions to the Cloud ", 27 September 2023
Explore the value of data architectures and learn how IBM’s database portfolio can help simplify data for all your applications, analytics and AI workflows.
Learn how an open data lakehouse approach can provide trustworthy data and faster analytics and AI projects execution.
Explore the data leader's guide to building a data-driven organization and driving business advantage.
Discover why AI-powered data intelligence and data integration are critical to drive structured and unstructured data preparedness and accelerate AI outcomes.
Gain unique insights into the evolving landscape of ABI solutions, highlighting key findings, assumptions and recommendations for data and analytics leaders.
Simplify data access and automate data governance. Discover the power of integrating a data lakehouse strategy into your data architecture, including cost-optimizing your workloads and scaling AI and analytics, with all your data, anywhere.
Explore how IBM Research is regularly integrated into new features for IBM Cloud Pak for Data.
Design a data strategy that eliminates data silos, reduces complexity and improves data quality for exceptional customer and employee experiences.
Watsonx.data enables you to scale analytics and AI with all your data, wherever it resides, through an open, hybrid and governed data store.
Unlock the value of enterprise data with IBM Consulting, building an insight-driven organization that delivers business advantage.

IMAGES
COMMENTS
Oct 16, 2021 · Essay Example: Introduction In the contemporary landscape of academic research, the management of data has emerged as a crucial component of the research process. As research endeavors become increasingly complex and data-intensive, the necessity of organizing, structuring, storing, and safeguarding
Nov 23, 2024 · Looking for a good essay, research or speech topic on Data Management? Check our list of 71 interesting Data Management title ideas to write about!
Jan 1, 2024 · Data Management Essay Topic Ideas & Examples. Data management is a crucial aspect of any organization's operations. It involves the collection, organization, analysis, and utilization of data to make informed decisions and drive business growth.
Why data management is important. Data management is a crucial first step to employing effective data analysis at scale, which leads to important insights that add value to your customers and improve your bottom line. With effective data management, people across an organization can find and access trusted data for their queries.
Data collection is a critical first step in the data management process and may be broadly classified as “primary data collection” (collection of data directly from the subjects specifically for the study) and “secondary use of data” (re-purposing data that was collected for some other reason – either for clinical care in the subject ...
Jan 29, 2022 · An advice on data protection and transfer, published at the website of Cardiff University, states that the data should be secured during the transit; sharing the personal data with other organizations should be conducted under the Data Protection Act; access to personal data must be restricted to the minimum number of the research participants, and the issues with identifiability should be ...
Aug 14, 2024 · Competitive Advantage: Proper data management entitles organisations to explore market trends, customer behaviours, and other insights that can help them outperform competitors. Data Management Responsibilities and Roles in IT industry. In the IT field, data management involves various job roles and responsibilities to process data properly.
On the one hand, Data Management essays we publish here precisely demonstrate how a really well-written academic piece of writing should be developed. On the other hand, upon your request and for an affordable price, a pro essay helper with the relevant academic experience can put together a high-quality paper model on Data Management from scratch.
Dec 9, 2022 · Top 6 benefits of data management. Data management is an essential part of data science, data analytics, and data engineering. Here are the top 6 benefits of data management: 1. Improved data quality. By effectively managing data, organizations can ensure that data errors are minimized, data duplication is avoided, and data integrity is maintained.
Jul 3, 2024 · While the data management tools for constructing generative AI applications are widely available, the data itself holds the value for both customers and businesses. High volumes of quality data must be properly organized and processed to successfully train models. This approach is a rapidly growing use case for modern data management.